big data
What is big data?
Big data is a combination of structured, semi-structured and unstructured data that organizations collect, analyze and mine for information and insights. It's used in machine learning projects, predictive modeling and other advanced analytics applications.
Systems that process and store big data have become a common component of data management architectures in organizations. They're combined with tools that support big data analytics uses. Big data is often characterized by the three V's:
- The large volume of data in many environments.
- The wide variety of data types frequently stored in big data systems.
- The high velocity at which the data is generated, collected and processed.
Doug Lany first identified these three V's of big data in 2001 when he was an analyst at consulting firm Meta Group Inc. Gartner popularized them after it acquired Meta Group in 2005. More recently, several other V's have been added to different descriptions of big data, including veracity, value and variability.
Although big data doesn't equate to any specific volume of data, big data deployments often involve terabytes, petabytes and even exabytes of data points created and collected over time.
Why is big data important and how is it used?
Companies use big data in their systems to improve operational efficiency, provide better customer service, create personalized marketing campaigns and take other actions that can increase revenue and profits. Businesses that use big data effectively hold a potential competitive advantage over those that don't because they're able to make faster and more informed business decisions.
For example, big data provides valuable insights into customers that companies can use to refine their marketing, advertising and promotions to increase customer engagement and conversion rates. Both historical and real-time data can be analyzed to assess the evolving preferences of consumers or corporate buyers, enabling businesses to become more responsive to customer wants and needs.
Medical researchers use big data to identify disease signs and risk factors. Doctors use it to help diagnose illnesses and medical conditions in patients. In addition, a combination of data from electronic health records, social media sites, the web and other sources gives healthcare organizations and government agencies up-to-date information on infectious disease threats and outbreaks.

Here are some more examples of how organizations in various industries use big data:
- Big data helps oil and gas companies identify potential drilling locations and monitor pipeline operations. Likewise, utilities use it to track electrical grids.
- Financial services firms use big data systems for risk management and real-time analysis of market data.
- Manufacturers and transportation companies rely on big data to manage their supply chains and optimize delivery routes.
- Government agencies use bug data for emergency response, crime prevention and smart city initiatives.
What are examples of big data?
Big data comes from many sources, including transaction processing systems, customer databases, documents, emails, medical records, internet clickstream logs, mobile apps and social networks. It also includes machine-generated data, such as network and server log files and sensor data from manufacturing machines, industrial equipment and internet of things devices.
In addition to data from internal systems, big data environments often incorporate external data on consumers, financial markets, weather and traffic conditions, geographic information, scientific research and more. Images, videos and audio files are forms of big data, too, and many big data applications involve streaming data that's processed and collected continually.
Breaking down big data V's: Volume, variety and velocity
Volume is the most cited characteristic of big data. A big data environment doesn't have to contain a large amount of data, but most do because of the nature of the data being collected and stored in them. Clickstreams, system logs and stream processing systems are among the sources that typically produce massive volumes of data on an ongoing basis.
In terms of variety, big data encompasses several data types, including the following:
- Structured data, such as transactions and financial records.
- Unstructured data, such as text, documents and multimedia files.
- semi-structured data, such as web server logs and streaming data from sensors.
Various data types must be stored and managed in big data systems. In addition, big data applications often include multiple data sets that can't be integrated upfront. For example, a big data analytics project might attempt to forecast sales of a product by correlating data on past sales, returns, online reviews and customer service calls.
Velocity refers to the speed at which data is generated and must be processed and analyzed. In many cases, big data sets are updated on a real- or near-real-time basis, instead of the daily, weekly or monthly updates made in many traditional data warehouses. Managing data velocity is becoming more important as big data analysis expands into machine learning and artificial intelligence (AI), where analytical processes automatically find patterns in data and use them to generate insights.
More characteristics of big data: Veracity, value and variability
Looking beyond the original three V's, other ones are often associated with big data. Including the following:
- Veracity. Veracity refers to the degree of accuracy in data sets and how trustworthy they are. Raw data collected from various sources can cause data quality issues that might be difficult to pinpoint. If they aren't fixed through data cleansing processes, bad data leads to analysis errors that can undermine the value of business analytics initiatives. Data management and analytics teams also need to ensure that they have enough accurate data available to produce valid results.
- Value. Some data scientists and consultants also add value to the list of big data's characteristics. Not all the data that's collected has real business value or benefits. As a result, organizations need to confirm that data relates to relevant business issues before it's used in big data analytics projects.
- Variability. Variability often applies to sets of big data, which might have multiple meanings or be formatted differently in separate data sources. These factors can complicate big data management and analytics.
Some people ascribe even more V's to big data; various lists have been created ranging from seven to 10.
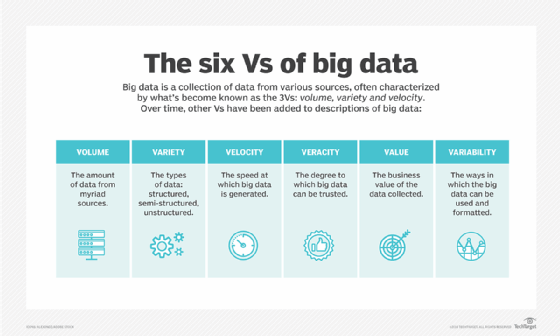
How is big data stored and processed?
Big data is often stored in a data lake. While data warehouses are commonly built on relational databases and contain only structured data, data lakes can support various data types and typically are based on Hadoop clusters, cloud object storage services, NoSQL databases or other big data platforms.
Many big data environments combine multiple systems in a distributed architecture. For example, a central data lake might be integrated with other platforms, including relational databases or a data warehouse. The data in big data systems might be left in its raw form and then filtered and organized as needed for particular analytics uses, such as business intelligence (BI). In other cases, it's preprocessed using data mining tools and data preparation software so it's ready for applications that are run regularly.
Big data processing places heavy demands on the underlying compute infrastructure. Clustered systems often provide the required computing power. They handle data flow, using technologies like Hadoop and the Spark processing engine to distribute processing workloads across hundreds or thousands of commodity servers.
Getting that kind of processing capacity in a cost-effective way is a challenge. As a result, the cloud is a popular location for big data systems. Organizations can deploy their own cloud-based systems or use managed big-data-as-a-service offerings from cloud providers. Cloud users can scale up the required number of servers just long enough to complete big data analytics projects. The business only pays for the data storage and compute time it uses, and the cloud instances can be turned off when they aren't needed.
How big data analytics works
To get valid and relevant results from big data analytics applications, data scientists and other data analysts must have a detailed understanding of the available data and a sense of what they're looking for in it. That makes data preparation a crucial first step in the analytics process. It includes profiling, cleansing, validation and transformation of data sets,
Once the data has been gathered and prepared for analysis, various data science and advanced analytics disciplines can be applied to run different applications, using tools that provide big data analytics features and capabilities. Those disciplines include machine learning and its deep learning subset, predictive modeling, data mining, statistical analysis, streaming analytics and text mining.
Using customer data as an example, the different branches of analytics that can be done with sets of big data include the following:
- Comparative analysis. This examines customer behavior metrics and real-time customer engagement to compare a company's products, services and branding with those of its competitors.
- Social media listening. This analyzes what people are saying on social media about a business or product, which can help identify potential problems and target audiences for marketing campaigns.
- Marketing analytics. This provides information that can be used to improve marketing campaigns and promotional offers for products, services and business initiatives.
- Sentiment analysis. All the data that's gathered on customer experience can be analyzed to reveal how they feel about a company or brand, customer satisfaction levels, potential issues and how customer service could be improved.
Big data management technologies
Hadoop, an open source distributed processing framework released in 2006, was initially at the center of most big data architectures. The development of Spark and other processing engines pushed MapReduce, the engine built into Hadoop, more to the side. The result is an ecosystem of big data technologies that can be used for different applications but often are deployed together.
IT vendors offer big data platforms and managed services that combine many of those technologies in a single package, primarily for use in the cloud. For organizations that want to deploy big data systems themselves, either on premises or in the cloud, various tools are available in addition to Hadoop and Spark. They include the following categories of tools:
- Storage repositories.
- Cluster management frameworks.
- Stream processing engines.
- NoSQL databases.
- Data lake and data warehouse platforms.
- SQL query engines.
Big data benefits
Organizations that use and manage large data volumes correctly can reap many benefits, such as the following:
- Enhanced decision-making. An organization can glean important insights, risks, patterns or trends from big data. Large data sets are meant to be comprehensive and encompass as much information as the organization needs to make better decisions. Big data insights let business leaders quickly make data-driven decisions that impact their organizations.
- Better customer and market insights. Big data that covers market trends and consumer habits gives an organization the important insights it needs to meet the demands of its intended audiences. Product development decisions, in particular, benefit from this type of insight.
- Cost savings. Big data can be used to pinpoint ways businesses can enhance operational efficiency. For example, analysis of big data on a company's energy use can help it be more efficient.
- Positive social impact. Big data can be used to identify solvable problems, such as improving healthcare or tackling poverty in a certain area.
Big data challenges
There are common challenges for data experts when dealing with big data. They include the following:
- Architecture design. Designing a big data architecture focused on an organization's processing capacity is a common challenge for users. Big data systems must be tailored to an organization's particular needs. These types of projects are often do-it-yourself undertakings that require IT and data management teams to piece together a customized set of technologies and tools.
- Skill requirements. Deploying and managing big data systems also requires new skills compared to the ones that database administrators and developers focused on relational software typically possess.
- Costs. Using a managed cloud service can help keep costs under control. However, IT managers still must keep a close eye on cloud computing use to make sure costs don't get out of hand.
- Migration. Migrating on-premises data sets and processing workloads to the cloud can be a complex process.
- Accessibility. Among the main challenges in managing big data systems is making the data accessible to data scientists and analysts, especially in distributed environments that include a mix of different platforms and data stores. To help analysts find relevant data, data management and analytics teams are increasingly building data catalogs that incorporate metadata management and data lineage functions.
- Integration. The process of integrating sets of big data is also complicated, particularly when data variety and velocity are factors.
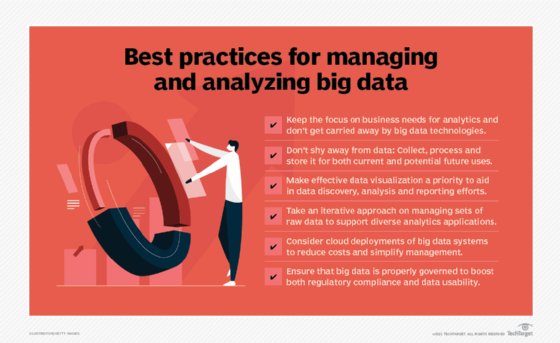
Keys to an effective big data strategy
Developing a big data strategy requires an understanding of business goals and the data that's available to use, plus an assessment of the need for additional data to help meet the objectives. The next steps to take include the following:
- Prioritizing planned use cases and applications.
- Identifying new systems and tools that are needed.
- Creating a deployment roadmap.
- Evaluating internal skills to see if retraining or hiring are required.
To ensure that big data sets are clean, consistent and used properly, a data governance program and associated data quality management processes also must be priorities. Other best practices for managing and analyzing big data include focusing on business needs for information over the available technologies and using data visualization to aid in data discovery and analysis.
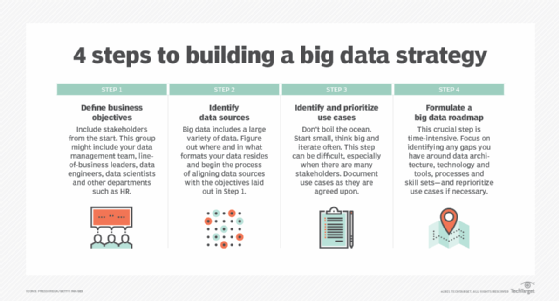
Big data collection practices and regulations
As the collection and use of big data have increased, so has the potential for data misuse. A public outcry about data breaches and other personal privacy violations led the European Union (EU) to approve the General Data Protection Regulation (GDPR), a data privacy law that took effect in May 2018.
GDPR limits the types of data organizations can collect and requires opt-in consent from individuals or compliance with other specified reasons for collecting personal data. It also includes a right-to-be-forgotten provision, which lets EU residents ask companies to delete their data.
While there isn't a similar federal law in the U.S., the California Consumer Privacy Act (CCPA) aims to give California residents more control over the collection and use of their personal information by companies that do business in the state. CCPA was signed into law in 2018 and took effect on Jan. 1, 2020. This bill was the first of its kind in the U.S. By 2023, 12 other states have enacted similar comprehensive data protection laws.
Other ongoing efforts to prevent technologies, such as AI and machine learning, from misusing big data include the EU's AI Act, which the European Parliament passed in March 2024. It's a comprehensive regulatory framework for AI use, providing AI developers and companies that deploy AI technology with guidance based on the level of risk an AI model poses.
To ensure that they comply with the laws that regulate big data, businesses need to carefully manage the process of collecting it. Controls must be put in place to identify regulated data and prevent unauthorized employees and other people from accessing it.
The human side of big data management and analytics
Ultimately, the business value and benefits of big data initiatives depend on the workers tasked with managing and analyzing the data. Some big data tools enable less technical users to run predictive analytics applications or help businesses deploy a suitable infrastructure for big data projects, while minimizing the need for hardware and distributed software know-how.
Big data can be contrasted with small data, a term that's sometimes used to describe data sets that can be easily used for self-service BI and analytics. A commonly quoted axiom is, "Big data is for machines; small data is for people."
The future of big data
A number of emerging technologies are likely to affect how big data is collected and used. The following tech trends will have the most influence on big data's future:
- AI and machine learning analysis. Large data sets are getting larger and thereby less efficiently analyzed by human eyes. AI and machine learning algorithms are becoming key to performing large-scale analyses and even preliminary tasks, such as data set cleansing and preprocessing. Automated machine learning tools are likely to be helpful in this area.
- Improved storage with increased capacity. Cloud storage capabilities are continually improving. Data lakes and warehouses, which can be either on-premises or in the cloud, are attractive options for storing big data.
- Emphasis on governance. Data governance and regulations will become more comprehensive and commonplace as the amount of data in use increases, requiring more effort to safeguard and regulate it.
- Quantum computing. Although less known than AI, quantum computing can also expedite big data analyses with improved processing power. It's in its early stages of development and only available to large enterprises with access to extensive resources.
If big data is becoming more of an issue for your organization, you'll want to know about these big data open source tools and technologies.