IBM (International Business Machines Corporation)
What is IBM (International Business Machines Corporation)?
IBM (International Business Machines Corporation) is a multinational technology company headquartered in Armonk, N.Y. It was founded in 1911 as the Computing-Tabulating-Recording Company (CTR) and was later renamed to IBM in 1924. IBM is one of the world's largest IT companies, with operations in over 170 countries.
IBM is known for its hardware and software products, including computers, servers, storage systems and networking equipment. It also provides consulting, technology and business services, such as cloud computing, data analytics and artificial intelligence (AI). IBM's research and development efforts have contributed to numerous technological innovations, including the development of the first programmable computer, the first hard drive and the first computer virus.
History of IBM
In its early years, IBM was widely associated with the punched card, invented by CTR employee Herman Hollerith. In 1914, Thomas J. Watson joined the company as general manager. CTR itself had been formed from three companies that sold grocery store scales, time recording devices and tabulators. Over the next few decades, Watson built the business machine company of the future, now known as International Business Machines.
In 1964, IBM introduced what was to become the de facto standard for large company business computers with its System/360. Orders for the mainframe computer line outpaced sales forecasts, and IBM received more than 1,000 orders within four weeks of the product's announcement, according to the company. System/360 ran the OS/360 operating system, but the successor OS Multiple Virtual Storage (MVS) became particularly influential.
MVS, which debuted in 1974, has been at the center of IBM's mainframe OS technology for more than 40 years. The OS for IBM's current-generation z Systems mainframes, z/OS, can trace its lineage to MVS. Over the decades, IBM has aimed to maintain forward compatibility as its mainframe OSes have evolved.
The popularity of System/360 and the subsequent mainframe models gave IBM an enduring market advantage, but the company faced several rivals in the mainframe space.
IBM also faced the challenge of minicomputers, or midrange systems, which were less expensive than mainframes and were targeted toward smaller businesses or departments within large enterprises. Digital Equipment Corp. and its Programmed Data Processor product line took off in the 1960s as well.
In 1981, IBM went small with the launch of the personal computer (PC). The IBM PC architecture soon became the standard for business use, and numerous vendors, including Compaq, soon entered the market with IBM-compatible PCs, also known as PC clones. IBM's selection of Microsoft Disk Operating System as the IBM PC's OS, and its subsequent adoption by IBM PC-compatible vendors, led to the dominance of Microsoft in the PC software market.
In the late 1980s, IBM added another hardware platform to its product mix: Unix workstations. IBM's RISC Technology system, eventually supplanted by the RISC System/6000, was the company's entry into the market, running IBM's implementation of Unix System V called Advanced Interactive eXecutive (AIX).
In 1985, IBM and Microsoft partnered to create a whole new OS for the PC called CP/DOS. It shipped in 1987 under the name OS/2. As Microsoft continued to develop Windows, the two companies split in 1990 with IBM carrying on OS/2 development. OS/2 was technically superior to Windows 3.1. But Microsoft had a hold on the PC vendors, and OS/2 was shunned. IBM ended development in 2001 and discontinued support in 2005.
As the 1990s dawned, IBM's core platforms included AIX, OS/2, MVS and OS/400.
While industry watchers questioned IBM's disparate platform strategy, IBM took steps to restructure its operations, granting more autonomy to its various product divisions in a bid to improve time to market. Under IBM CEO and Chairman John Akers, the restructuring program hit a wall, and the company announced a $5 billion loss for calendar year 1992. The loss was attributed, in part, to the restructuring initiative's costs, which included early retirements and the shuttering of production lines.
Amid the financial struggle, IBM began its push into IT services. The services push was led by executive Sam Palmisano, who would go on to become CEO of the company years later. The company captured a watershed data center outsourcing contract with Eastman Kodak Co. in 1989. Two years later, IBM Global Services (now IBM Consulting) was launched as the focal point of Big Blue's -- IBM's nickname -- IT services business.
IT outsourcing became an important element of IBM's services enterprise, as did management consulting. IBM cemented its role in the latter service with its $3.5 billion acquisition of PricewaterhouseCooper's management consulting arm, PwC Consulting, in 2002.
As it happens, IBM tapped the management consulting ranks to run the company and hired Louis Gerstner, a former McKinsey & Co. consultant and chairman of RJR Nabisco, to become IBM CEO in 1993. Under Gerstner, IBM continued its service push, in addition to expanding its role in software. In 1995, IBM acquired Lotus Development Corp. and then merged with Tivoli Systems Inc. the following year.
While software and services grew in importance, IBM looked to shed some of its hardware operations. IBM CEO Sam Palmisano, who replaced Gerstner and had earlier led IBM Global Services, began divesting the firm of commodity products. This divestment culminated in 2005 with the sale of its PC division to China's Lenovo Group. The two companies inked a strategic alliance in which IBM became Lenovo's preferred services provider.
By the late 2000s, IBM's hardware sales accounted for just 10% of total revenue, while services and consulting accounted for two-thirds of revenue, with software and miscellaneous (financing) bringing in the rest. IBM continued an aggressive acquisitions strategy in the new century, but it was all software and services firms.
In the 2010s, IBM began recasting itself as a cognitive solutions and cloud computing platform company. Virginia "Ginni" Rometty, appointed IBM CEO in 2011, emphasized a core group of strategic imperatives for IBM: analytics, cloud, mobility and security.
In 2013, IBM acquired SoftLayer Technologies, a Texas-based cloud service provider (CSP). This gave IBM a jump-start to its cloud strategy, which was lagging well behind Amazon Web Services (AWS), Microsoft and Google. IBM has added facilities and called its CSP business IBM Cloud.
In July 2019, IBM made its biggest purchase ever, spending $34 billion to acquire leading Linux-provider Red Hat Software. It merged Red Hat's hybrid cloud OpenStack software with IBM Cloud and on-premises services for a hybrid cloud approach to digital transformation.
IBM divestitures
IBM made some notable divestitures in recent years. In 2015, IBM sold off its x86 server business to Lenovo Group. Lenovo has since become the world's largest x86 vendor, owing primarily to sales in its native China.
In 2020, IBM announced the spinoff of its managed IT infrastructure business from the Global Technology Services unit. Managed services were primarily for on-premises IT infrastructure, which has been losing favor among enterprises as they move to the cloud. The unit was spun off as a standalone company called Kyndryl with 90,000 employees and annual sales of $19 billion.
In 2021, IBM began shopping around its Watson Health healthcare business and sold off the data assets to private equity firm Francisco Partners. Terms of the deal were not disclosed, but it was believed to be around $1 billion.
IBM products and services
Server hardware
Five decades since the launch of IBM System/360, the company continues to sell mainframe-class computers. IBM positions its z Systems product line as enterprise infrastructure for its customers' cognitive business. IBM targets a range of solutions for its z Series products, including analytics, blockchain, cloud and DevOps.
Meanwhile, the company aims its Power Systems enterprise servers toward big data and analytics applications. Power Systems run IBM's AIX and IBM i OSes, as well as Linux. In another nod to open source, IBM introduced its LinuxONE system as a hardware platform using its mainframe hardware but running several Linux enterprise distributions.
Storage
On the hardware side, IBM offers products including its FlashSystem all-flash arrays, Storwize systems and other hybrid arrays, Fibre Channel storage area network hardware, storage media and tape products. The company is making a push into software-defined storage with its Spectrum Storage Suite and Cleversafe object storage technology.
IBM also remains an enthusiastic supporter of tape backup storage. It touts two clear advantages of tape: massive storage capacity in the exabytes and airbrakes in which tape sits in a storage rack disconnected from the network. Hackers can't get to something that isn't connected, and an airbrake is the ultimate hacker defense.
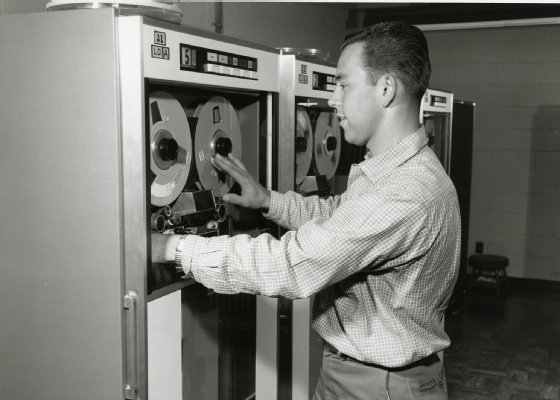
Software
IBM's varied software line includes analytics offerings, such as IBM Cognos Analytics, IBM Statistical Product and Service Solutions (SPSS), IBM Maximo Asset Management and Db2. Many of IBM's products in this field came through acquisition: The company purchased Maximo in 2006, Cognos in 2008 and SPSS in 2009.
IBM also provides IT infrastructure software, including its WebSphere Application Server and MQ messaging middleware.
The company's software lineup in the mobile and social space includes the IBM Verse (now HCL Verse) business email offering and the IBM Notes (now HCL Notes) collaboration product. In addition, IBM's security software includes MaaS360 for mobile device security and IBM QRadar Security Intelligence Platform, a security information and event management product.
IBM customers may acquire software licenses through Passport Advantage, the company's licensing program for larger enterprises, or Passport Advantage Express, a program designed for medium-sized businesses. Fix Central, meanwhile, is an element of IBM support that provides fixes and updates for IBM customers' software and OSes. Fix Central provides hardware support as well.
IBM was an early adopter of the Linux OS, supporting clinics on its mainframes as early as 1999. Currently, it supports the enterprise versions of Red Hat Linux, SuSe Linux and Canonical (Ubuntu) on its z Series of mainframes, LinuxONE server and its Power-based servers.
Services
IBM's service units include Global Business Services, which houses Big Blue's management consulting operations, and Global Technology Services, which provides mobility, networking, business continuity and outsourcing, among other services. Like other large IT services providers, in recent years, IBM has moved to purchase companies offering cloud consulting and implementation services. In 2016, for example, IBM purchased Bluewolf, a Salesforce channel partner and cloud consultant. Bluewolf was folded into IBM's Interactive Experience practice, which is part of Global Business Services. In 2015, IBM acquired Meteorix LLC, a Workday services partner.
Cloud
IBM's SmartCloud software and services offering got off the ground in 2011. That move was followed in 2013 by IBM's acquisition of SoftLayer Technologies Inc., an infrastructure-as-a-service provider. Following that deal, SmartCloud and SoftLayer were grouped together in a cloud services division.
Since then, however, IBM has coalesced its cloud services offerings around its Bluemix platform-as-a-service offering. As of fall 2016, Bluemix had incorporated SoftLayer cloud products and services into a broader portfolio of infrastructure, platform and application services. In October 2017, IBM then rebranded its entire cloud portfolio as IBM Cloud. IBM's more integrated cloud offering competes against such rivals as AWS, Google and Microsoft.
One area of cloud computing that IBM is focused on more so than its competitors is bare-metal computing. In bare-metal computing, only hardware is provided, and an operating environment must be manually installed. In a typical cloud service from AWS or Microsoft, they provide either Linux or Windows operating environments.
The advantage of bare metal is that it enables a customer to keep its custom or older operating environment. It enables customers to do a lift and shift, where the installation of OS, apps and data that have all been operating in the current data center occurs without need for modification.
Cognitive offerings
The IBM Watson supercomputer, which pulls together AI and analytical software, is the company's flagship cognitive computing offering. A number of technologies and discrete products have spun out of IBM's cognitive computing system and its related research. Customers, for example, can use Watson application programming interfaces (APIs) to embed cognitive computing components into their applications. IBM also offers products with built-in cognitive capabilities. Those offerings include IBM Watson Internet of Things and Watson Analytics for Social Media. IBM has looked to use its cloud technology as it rolls out Watson-related products. Watson APIs, for example, are available via Bluemix.
Research and development
Thomas J. Watson Jr., who succeeded his father as IBM CEO in 1956, put the company on a research and development track. Big Blue's research center, launched in 1961, encompasses research labs in Yorktown Heights, N.Y., and Cambridge, Mass., as well as an industry solution lab in Hawthorne, N.Y. Notable developments out of IBM's research center include the invention of dynamic RAM and the Fortran language. More recent research endeavors include blockchain, quantum computing and cognitive technology.
IBM also invests heavily in semiconductor research as it investigates chips to support cloud computing and big data systems. But, while Big Blue conducts research on silicon, the company exited the microelectronics manufacturing business in 2015, selling that operation to GlobalFoundries.