8 top generative AI tool categories for 2025
Need a generative AI-specific tool for your organization's development project? Explore the major categories these tools fall into and their capabilities.
The popularity of generative AI services such as ChatGPT has galvanized interest in applying these new tools to practical enterprise applications. Nowadays, virtually every enterprise app is enhanced with generative AI capabilities.
Most AI, data science, machine learning development, deployment and operational tools support generative AI use cases. Various flavors of these tools help manage the AI development lifecycle, govern data for AI development, and mitigate security and privacy risks. Although these capabilities can also be used to improve generative AI development, this article focuses on generative AI-specific tools.
There are also many emerging types of generative AI that don't use such large language models (LLMs) for tasks like generating images, video, audio, synthetic data and translating across languages. This includes models built using generative adversarial networks, diffusion, variational autoencoders and multimodal techniques.
With that in mind, here are more details about the top categories of generative AI development tools and their capabilities, along with some of the leading vendor or open source implementations. It's also worth noting that many of the leading vendors are starting to expand their core offerings to support multiple categories through acquisition or development. While many existing tools support a specific category, enterprises might want to explore top platform capabilities that support these features directly or via integrated marketplaces when planning their generative AI strategy.
This article is part of
What is GenAI? Generative AI explained
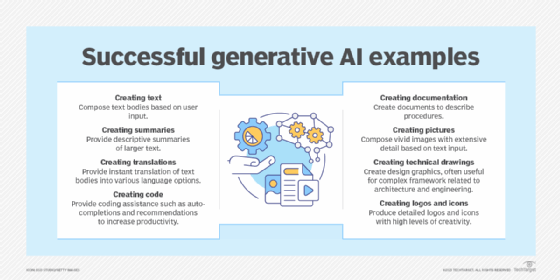
1. Foundation models and services
For the most part, new generative AI tools have focused on simplifying the development and responsible use of LLMs built using transformer approaches pioneered by Google researchers in 2017. New foundation models built on LLMs can often be used directly out of the box to enhance existing applications. In other cases, vendors are developing domain-specific models for various industries and use cases. Examples of top foundation models and services include Anthropic's Claude, Baidu Research's Ernie, Cohere's Command, Facebook's Llama series, Google's Gemini, Microsoft's Orca, OpenAI's GPT series and Technology Innovation Institute's Falcon LLM. Top domain-specific LLMs include C3 AI; DeepMind's AlphaCode; Google's Med-Palm; Nvidia's NeMo, BioNeMo and Picasso; and OpenAI's Codex.
2. Cloud generative AI platforms
The major cloud platforms are also rolling out a suite of generative AI capabilities to help enterprises develop, deploy and manage generative AI models and capabilities. Cloud provider generative AI platforms include AWS Generative AI, Google Generative AI, IBM Watsonx and Microsoft Azure AI Fundamentals. Popular third-party generative AI development platforms to develop generative AI in the cloud include offerings from Hugging Face and Nvidia.
3. Use case optimization tools
General-purpose foundation models can generate authoritative-sounding and well-articulated text. They also have a propensity to hallucinate and generate inaccurate information. Generative AI-specific development tools can also help enterprises roll their own LLMs that are tuned to their unique needs and expertise. Retrieval-augmented generation (RAG) can prime foundation models to improve accuracy. Fine-tuning tools help enterprises calibrate foundation models. RAG and fine-tuning tools are sometimes used together to balance gains from each approach. Top RAG tools include ChatGPT Retrieval Plugin, Hugging Face Transformers plugin and open source tools such as Farm, Haystack and Realm. Fine-tuning capabilities are baked into services to access most commercial foundation models. Additional third-party fine-tuning tools include Entry Point and Synthflow (formerly Fine-Tuner), as well as products from Hugging Face that work with open source models.
4. Quality assurance and hallucination mitigation tools
New hallucination detection tools can help identify and reduce the prevalence of hallucination for various use cases. Top hallucination mitigation tools include Galileo's Evaluation Intelligence Platform, Gleen, TruLens and Vectara's Vectara platform. New research techniques, such as the Woodpecker algorithm, could help enterprises interested in developing their own hallucination mitigation workflows. Many of these vendors have released open source variants of these, including Galileo's Luna suite of evaluation foundation models, open source TruLens (now owned by Snowflake) and Vectara's Hughes Hallucination Evaluation Model.
5. Prompt engineering tools
Prompt engineering tools help manage interactions with LLMs and testing of LLMs. User-oriented variants of these tools make it easier to develop and manage libraries of useful prompts. Engineering-oriented variations help automate testing processes to identify bias, toxicity or hallucinations. Top prompt engineering tools include BetterPrompt, OpenPrompt, PromptAppGPT, Prompt Engine and Promptimize.
6. Data aggregation tools
Early foundation models supported limited context windows that characterize how much data an LLM can process in one query. Although these models are getting better at handling larger context windows, developers have crafted various types of tools to work with larger data sets. Data chaining tools like LangChain and Dust can automate the process of inputting multiple documents into LLMs. Vector databases store data in an intermediate format, called an embedding space, to work with LLMs. Top vector databases to consider include Chroma, Faiss, Pinecone Systems' Pinecone, Qdrant and Weaviate.
7. Agentic and autonomous AI tools
Developers are also exploring ways to automate interactions across one or more foundation models and the back-end services to which they might relate. In the long run, this could help enable the development of agentic AI or autonomous AI. Autonomous and agentic AI tools include open source tools like AgentGPT, AutoGPT, BabyAGI and OthersideAI's self-operating computer framework. Platform vendors are also rolling out new services to integrate workflows across multiple LLM models and services.
8. Generative AI cost optimization tools
AI cost optimization tools help strike the best balance among performance, accuracy and cost. These tools are still early in their development, but one early example is Martian's Model Router. Existing cloud cost optimization leaders will likely develop related offerings in the long run.
George Lawton is a journalist based in London. Over the last 30 years he has written more than 3,000 stories about computers, communications, knowledge management, business, health and other areas that interest him.