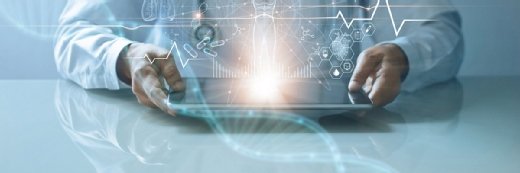
Getty Images/iStockphoto
How generative AI could change healthcare
Generative AI has joined the ranks of healthcare professionals in early use cases from medical research to patient communications. AI at scale isn't far behind.
The healthcare sector has seized on the transformative potential of generative AI, throwing millions of dollars into exploring practical applications.
Some 75% of leading healthcare companies are already experimenting with generative AI or planning to scale generative AI across the enterprise, according to the "2024 Life Sciences and Health Care Generative AI Outlook Survey" from the Deloitte Center for Health Solutions.
Meanwhile, the industry's investments in generative AI are expected to increase 17-fold within a decade. Market research firm Market.us estimated that the global generative AI in healthcare market will grow to $17.2 billion in 2032, up from $800 million in 2022.
"We're starting to see early use cases," said Jeff Wong, global chief innovation officer at professional services firm EY.
Groups of doctors and hospital systems are figuring out how to use generative AI. For example, Wong and other experts said healthcare professionals are adopting generative AI to summarize research and clinical notes, and even to aid in diagnosing patients.
"We're seeing a ton of work with people on the ground figuring out how they can use this tool to do their jobs better," Wong added.
But this early in adoption, healthcare companies need to review the most relevant generative AI use cases for a sector where lives are at risk.
6 top use cases for generative AI in healthcare
According to health tech experts, generative AI is poised to affect research capabilities, clinical decision support, patient care, healthcare administration, communication, and clinician education and training.
1. Research
Medical research is already benefiting from generative AI, and experts expect the technology to both expand and accelerate research in the coming years.
For example, generative AI can create synthetic data, said Ivo Dinov, an expert from the University of Michigan who, among other roles, is a professor of computational medicine and bioinformatics and associate director for education and training at the Michigan Institute for Data Science.
Dinov said this use of generative AI creates a significant advantage in research, where certain types of data -- such as imaging scans of the brain -- are expensive to produce and limited in number. Using generative AI to create synthetic data gives researchers access to the population-size pool of data required for optimal research results.
Generative AI also offers new capabilities during drug discovery, said Ashkan Afkhami, global leader for the healthcare practice at BCG X, Boston Consulting Group's digital build and analytics division. The technology can assist throughout the drug discovery process, from helping design new compounds to selecting drug trial participants to optimizing searches through vast volumes of research data.
Generative AI's use in research could reduce the cost and time required for breakthroughs by 20% to 30%, according to Afkhami. "It's one area where we're seeing really great results, and they're pretty meaningful," he said.
2. Clinical decision support
Classic AI and other intelligent technologies have helped clinicians treat their patients for years, but generative AI is bringing new capabilities to steps such as diagnostics and disease management.
Only 11% of clinical decisions today are assisted by generative AI tools, according to the 2023 "Clinician of the Future" report from Elsevier Health, a scientific publisher and data analytics company. Generative AI can go beyond the capabilities of other AI and machine learning systems in how it analyzes unstructured data and generates questions for clinicians to ask patients.
"Existing tools are more binary than generative AI," Afkhami said. Compared with established tools, generative AI can parse more data from places such as electronic health records and more effectively distinguish factors such as age, gender, family history and personal history to generate more personalized results.
3. Patient care
Generative AI also can aid clinicians in patient care and clinical decision-making. For example, it can offer enhanced medical image reading, assisted disease diagnosis and more personalized therapeutic plans.
Generative AI currently is, or soon will be, affecting patient care, said Shannon Germain Farraher, a senior analyst in healthcare digital strategy and innovation at Forrester Research. Generative AI could help clinicians prioritize patient needs, for example, or explore social determinants of health to address individual as well as population health issues.
Many patients see the potential of generative AI, too, according to the "2023 Health Care Consumer Survey" from Deloitte's Center for Health Solutions. That research found that 53% of the responding 2,014 U.S. adult participants believe generative AI could improve access to healthcare -- for example, through using the technology to learn about conditions and treatment options -- and 46% believe it could make healthcare more affordable.
4. Healthcare administration
Generative AI is also expected to reshape healthcare administration. Software vendors are developing generative AI-based tools that can perform many of the routine tasks that workers throughout the healthcare ecosystem -- from clinicians to support staff to payers -- now handle, Farraher said.
As an example, she said, generative AI capabilities can support clinical documentation capture and summarize information from recordings of patient visits. This enables clinicians to focus more on the patient and less on note taking.
Furthermore, generative AI could pre-populate healthcare documents and prior authorization forms. It also could handle certain patient requests for information more effectively than the existing automation used in healthcare contact centers and administrative offices.
In addition to saving time, experts said generative AI's use for administration in the healthcare system could yield improvements.
Much of healthcare is governed by policies. Michael Louis Burns, assistant professor of anesthesiology and assistant director of informatics and data analytics at the University of Michigan, talked about using generative AI to search, summarize and analyze the significant volume of policies that exist within a typical medical center or healthcare system.
Burns, who is also a member of the University of Michigan's E-Health and Artificial Intelligence initiative, said such analysis by generative AI could identify outdated information, gaps in procedures or conflicts within those policies. "You can ask generative AI, 'What needs to be updated?' and find that information and get it into the right hands quickly," he said.
5. Communication
Some experts see generative AI -- and more specifically, tools built on large language models such as ChatGPT -- supporting the various communication requirements within the medical industry.
Afkhami listed some potential applications. Medical technology, pharmaceutical, biopharma and medical device companies could generate content and copy required for marketing and sales initiatives. They could also use generative AI to create instructions and other information for customers.
"This is an area where we're seeing organizations go from a three- to six-month process down to three to four weeks," he said.
In addition, healthcare entities from drugmakers to hospitals can generate patient information such as treatment instructions or hospital discharge notes, Afkhami said. Such use cases not only save healthcare professionals time, but also have the potential to improve the quality of care.
Afkhami explained that healthcare professionals can use generative AI tools to dynamically change the content they're providing and tailor information to each patient. For example, a hospital could break down instructions thoroughly for patients with limited literacy skills or add more pictures or audio options.
"That's the power we're talking about: You can create multimodal engagement models," he said.
As with other uses of generative AI, Afkhami stressed strong oversight to ensure the information generated is accurate, complete and understandable.
6. Education and training
Education and training is another area where generative AI could change healthcare.
For example, generative AI can produce personalized homework, assessment scenarios and exam questions for students and trainees. These materials support students' learning, concept mastery and ability to demonstrate knowledge.
"I can put in a 900-page textbook and ask [a generative AI tool] to generate five homework questions for students to demonstrate their knowledge," Dinov said. "It pulls in and understands the content, it generates unique questions that are rational and based on the syllabus for the class, and it can then test the validity of the student responses."
In Dinov's view, using generative AI for training and education could also extend knowledge and create more equity for providers and patients.
"Think about the population in the world who aren't in enriched environments," he said. "They can get access to AI systems that can train them, and it can help us reach out to train them outside of traditional academic settings. So it can expand opportunities."
Generative AI's risks in healthcare
Although generative AI's potential in the healthcare sector is immense, so are the potential risks of using the technology where people's well-being and lives could be at stake. Consequently, health tech experts stressed the need for strong governance and oversight of generative AI's use in the medical space.
"The stakes are higher here [than in other industries], and we have to be thoughtful about that," Wong said. "There are regulatory issues here, but also the nature of healthcare in caring for people and their health and lives is a factor of concern."
Potential risks that need attention include the following:
- Privacy concerns. Generative AI, like all AI, requires data. To use this technology in healthcare, AI systems need patient data. That raises significant privacy concerns and security issues, Afkhami said, noting that the U.S.'s HIPAA and other regulations impose strict privacy and security requirements on such data. Any use of generative AI must adhere to those rules.
- Bias. Generative AI's results, or output, are only as accurate as its training data. If a tool's training data is incomplete or biased, then the model's outputs will also be biased, Wong explained.
- Inaccurate and made-up results. In addition to biased results, generative AI sometimes produces inaccurate results and made-up answers known as hallucinations. These represent some of the most significant risks of generative AI in healthcare, given the potential for catastrophic results due to clinical actions based on inaccurate outputs or outright hallucinations.
- Costs. U.S. healthcare costs drive significant and often bitter policy debates. Generative AI has the potential to reduce costs, experts said. But they also noted that generative AI itself requires significant resources -- including time, talent and money -- to develop, train, tune, manage and update. As a result, there are questions about whether all medical entities that could benefit from generative AI will have the ability to pay for it.
- An even greater divide in access to quality care. Generative AI as well as other forms of AI and machine learning have already improved healthcare in many ways and will continue to do so. But there might be uneven access to such improvements due to various factors, including the costs associated with the technologies, Dinov said.
Mary K. Pratt is an award-winning freelance journalist with a focus on covering enterprise IT, cybersecurity management and strategy.