RFM analysis (recency, frequency, monetary)
What is RFM analysis (recency, frequency, monetary)?
RFM analysis is a marketing technique used to quantitatively rank and group customers based on the recency, frequency and monetary total of their recent transactions to identify the best customers and perform targeted marketing campaigns. The system assigns each customer numerical scores based on these factors to provide an objective analysis. RFM analysis is based on the marketing adage that "80% of your business comes from 20% of your customers."
RFM analysis ranks each customer on the following factors:
- Recency. How recent was the customer's last purchase? Customers who recently made a purchase will still have the product on their mind and are more likely to purchase or use the product again. Businesses often measure recency in days. But, depending on the product, they may measure it in years, weeks or even hours.
- Frequency. How often did this customer make a purchase in a given period? Customers who purchased once are often more likely to purchase again. Additionally, first time customers may be good targets for follow-up advertising to convert them into more frequent customers.
- Monetary. How much money did the customer spend in a given period? Customers who spend a lot of money are more likely to spend money in the future and have a high value to a business.
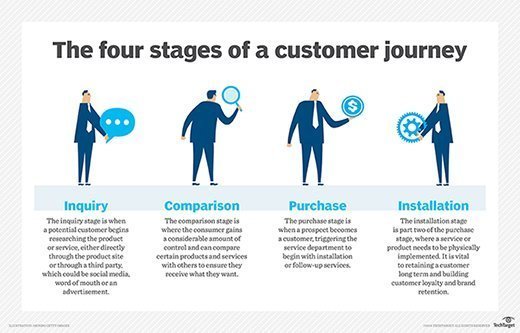
RFM analysis stands as a cornerstone marketing technique, empowering businesses to rank and group customers based on their transaction history to optimize marketing strategies.
How RFM analysis works
RFM analysis scores customers on each of the three main factors. Generally, a score from 1 to 5 is given, with 5 being the highest. Various implementations of an RFM analysis system may use slightly different values or scaling, however.
The collection of three values for each customer is called an RFM cell. In a simple system, organizations average these values together, then sort customers from highest to lowest to find the most valuable customers. Some businesses, instead of simply averaging the three values, weigh the values differently.
For example, a car dealership may recognize that an average customer is highly unlikely to buy several new cars in a timeframe of just a few years. But a customer who does buy several cars -- a high-frequency customer -- should be highly sought after. So, the dealership may choose to weigh the value of the frequency score accordingly.
RFM analysis is also valuable for organizations that do not sell products directly to customers. Nonprofits and charities can use RFM analysis to find the best donors, for example, as those who have donated in the past are more likely to donate again in the future.
Lastly, businesses that do not rely on direct payments from customers may use different factors in their analysis. For example, websites and apps that value readership, number of views or interaction may use an engagement value instead of monetary value to perform an RFE (recency, frequency, engagement) analysis instead of a standard RFM analysis using the same techniques as the latter. We'll touch more on RFE in a later section.
Segmentation of customers in RFM analysis
RFM analysis is a powerful tool in marketing that helps marketers make the best of their advertising budget.
Instead of simply using an overall RFM average value to identify the best customers, businesses can use RFM analysis to identify clusters of customers with similar values. Called customer segmentation, this process is used to produce targeted direct marketing campaigns tailored to specific customer types. It enables businesses to use email or direct mail marketing to target messages that a large swath of specific kinds of customers are more likely to respond to.
Some examples of customer types include the following:
- Whales. The biggest customers with high (5,5,5) values in all three factors that should be targeted with special promotions to keep them active.
- New customers. Customers with high recency and low frequency (5,1,X) are new customers. A targeted follow-up may convert them into repeat customers.
- Lapsed customers. Customers with low recency but high value (1,X,5) were once valuable customers but have since stopped. A targeted message may reactivate them.
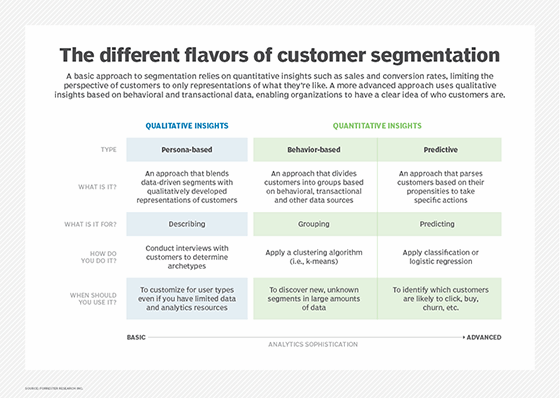
Businesses have various tools at their disposal to segment customers for RFM analysis and other kinds of business intelligence (BI) analytics methods.
How to perform RFM analysis
The landscape of data analysis has evolved, offering sophisticated tools beyond the traditional Excel and Power Pivot from Microsoft. Modern BI tools like Tableau and Google Analytics provide dynamic capabilities for e-commerce segmentation, while advanced CRM systems now come with built-in analytics features.
These tools facilitate a deeper dive into customer data, allowing for a more nuanced RFM analysis with intuitive visualizations and real-time insights.
The following checklist serves as a general example of how an organization can get started conducting an RFM analysis:
- Data collection. Gather comprehensive transaction data for each customer.
- Score assignment. Use modern BI tools to assign scores for recency, frequency and monetary values.
- Segmentation. Apply AI and machine learning (ML) tools to automate the segmentation process based on RFM scores.
- Analysis. Utilize advanced analytics to interpret the segmentation, identifying key customer groups.
- Strategy development. Craft tailored marketing strategies for different customer segments identified through RFM analysis.
- Compliance check. Ensure all RFM analysis practices comply with relevant data privacy regulations.
- Action. Implement targeted marketing campaigns based on the analysis.
- Review and adjust. Continuously monitor the results of the business's strategies and adjust based on performance and changing customer behaviors.
Navigating data privacy regulations in RFM analysis
The importance of adhering to data privacy regulations such as the General Data Protection Regulation (GDPR) and the California Consumer Privacy Act (CCPA) cannot be overstated in RFM analysis. Businesses must ensure that their data handling and analysis practices comply with these laws to protect customer information.
This includes obtaining consent for data collection, ensuring transparency in data processing and providing customers with control over their data. Adapting RFM analysis to operate within these legal frameworks is crucial for maintaining customer trust and avoiding regulatory penalties.
Addressing the limitations of RFM analysis
Using RFM modeling can provide valuable insights about customers. But it does not take into account many other factors about the customer.
In-depth targeted marketing may also use the type of item purchased or customer campaign responses as factors. Customer demographics such as age, sex and ethnicity are not covered in RFM analysis either. Additionally, RFM only uses historical data about customers and may not predict future customer activity.
Predictive methods may be able to identify future customer behavior that RFM analysis cannot. For example, AI and ML technologies, as mentioned previously, are revolutionizing RFM analysis by automating the segmentation process and enabling more accurate predictions of future customer behavior.
These technologies can uncover subtle patterns in customer data, providing insights that go beyond manual analysis. By using AI, businesses can not only categorize customers more efficiently but also anticipate their future actions, tailoring marketing strategies to meet evolving customer needs.
Furthermore, measuring engagement beyond the transaction is critical. This includes measuring social media interactions, app usage and website visits. Recognizing this, the RFE analysis emerges as a powerful alternative for businesses focusing on digital channels.
Integrating digital engagement metrics with traditional RFM factors offers a comprehensive view of customer behavior, enabling businesses to craft strategies that resonate with the digitally savvy customer.
Actionable strategies post-RFM analysis
After segmenting customers through RFM analysis, the focus shifts to engaging these distinct groups with strategies designed to maximize value and foster long-term loyalty. The insights garnered from RFM analysis provide a nuanced understanding of customer behaviors and preferences, which is instrumental in crafting personalized marketing strategies.
For high-value customers, often referred to as whales, businesses can design exclusive offers or access to premium services that acknowledge their importance to the brand. This could include early access to new products, exclusive membership benefits or personalized services that cater to their specific needs and preferences. These gestures of recognition not only enhance the customer experience but also reinforce the customer's decision to engage with the brand, thereby increasing their lifetime value.
For customers identified as having potential for increased engagement, such as those with high recency but lower frequency and monetary scores, targeted communication strategies can be employed to elevate their transaction levels.
This might involve tailored email marketing campaigns that highlight products or services specifically aligned with their past purchasing behavior, coupled with incentives like discounts or loyalty points to encourage repeat purchases. Additionally, using social media and other digital platforms to create interactive and engaging content can further draw these customers into a closer relationship with the brand.
Reengagement campaigns for lapsed customers, identified by low recency scores, require a careful approach that often involves understanding the reasons behind their decreased engagement. Surveys or feedback requests can provide valuable insights into their experiences and what might entice them back.
Based on this information, personalized reengagement offers that address any concerns or barriers they've experienced can be particularly effective. For instance, if price sensitivity is a common theme among lapsed customers, reintroduction offers or price-match guarantees might reignite their interest.
It's crucial that these strategies not only aim to reactivate but also to understand and address the underlying factors contributing to their lapse, ensuring any reengagement is sustainable over the long term.
The key lies in the personalized approach, ensuring that each customer segment receives communication and offers that resonate with their specific behaviors and preferences, ultimately leading to increased loyalty and lifetime value.
While personalization and segmentation use different kinds of customer data, both benefit marketing teams. Find out the main differences between personalization and segmentation.