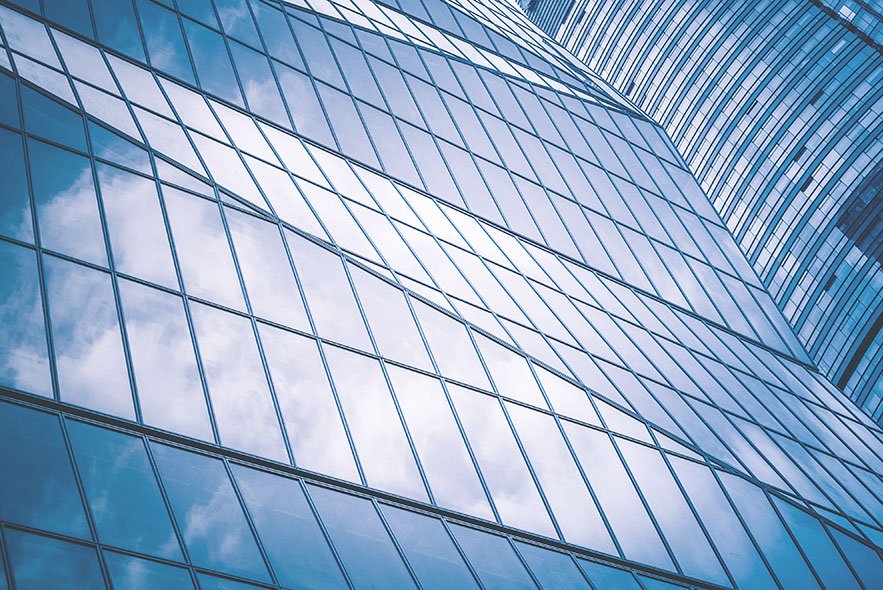
What is responsible AI?
Responsible AI is an approach to developing and deploying artificial intelligence (AI) from both an ethical and legal point of view. The goal of responsible AI is to use AI in a safe, trustworthy and ethical fashion. Using AI responsibly should increase transparency and fairness, as well as reduce issues such as AI bias.
Proponents of responsible artificial intelligence believe a widely adopted governance framework of AI best practices makes it easier for organizations to ensure their AI programming is human-centered, interpretable and explainable. However, ensuring trustworthy AI is up to the data scientists and software developers who write and deploy an organization's AI models. This means the steps required to prevent discrimination and ensure transparency vary from company to company.
Implementation also differs among companies. For example, the chief analytics officer or other dedicated AI officers and teams might be responsible for developing, implementing and monitoring the organization's responsible AI framework. Organizations should have an explanation on their website of their AI framework that documents its accountability and ensures the organization's AI use isn't discriminatory.
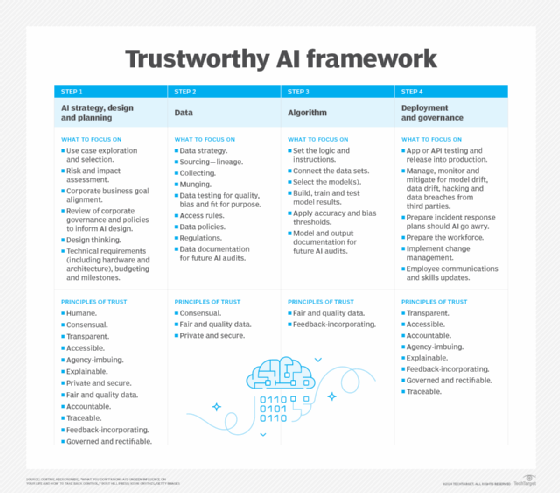
Why responsible AI is important
Responsible AI is an emerging area of AI governance. The use of the word responsible is an umbrella term that covers both ethics and AI democratization.
This article is part of
What is enterprise AI? A complete guide for businesses
Often, the data sets used to train machine learning (ML) models used in AI introduce bias. Bias gets into these models in one of two ways: incomplete or faulty data, or from the biases of those training the ML model. When an AI program is biased, it can end up negatively affecting or hurting humans. For example, it can unfairly decline applications for financial loans or, in healthcare, inaccurately diagnose a patient.
As software programs with AI features become more common, it's apparent there's a need for standards in AI beyond the three laws of robotics established by science fiction writer Isaac Asimov.
The implementation of responsible AI can help reduce bias, create more transparent AI systems and increase user trust in those systems.
What are the principles of responsible AI?
AI and machine learning models should follow a list of principles that might differ from organization to organization.
For example, Microsoft and Google both follow their own list of principles. In addition, the National Institute of Standards and Technology (NIST) has published a 1.0 version of its Artificial Intelligence Risk Management Framework that follows many of the same principles found in Microsoft and Google's lists. NIST's seven principles include the following:
- Valid and reliable. Responsible AI systems should be able to maintain their performance in different and unexpected circumstances without failure.
- Safe. Responsible AI must keep human life, property and the environment safe.
- Secure and resilient. Responsible AI systems should be secure and resilient against potential threats, such as adversarial attacks. Responsible AI systems must be built to avoid, protect against and respond to attacks, while also being able to recover from them.
- Accountable and transparent. Increased transparency is meant to build trust in the AI system, while making it easier to fix problems associated with AI model outputs. This principle requires that developers take responsibility for their AI systems.
- Explainable and interpretable. Explainability and interpretability are meant to provide in-depth insights into the functionality and trustworthiness of an AI system. For example, explainable AI tells users why and how the system got to its output.
- Privacy-enhanced. The privacy principle enforces practices that safeguard end-user autonomy, identity and dignity. Responsible AI systems must be developed and deployed with values, such as anonymity, confidentiality and control.
- Fair with harmful bias managed. Fairness focuses on eliminating AI bias and discrimination. It attempts to ensure equality and equity, a difficult task as these values differ among organizations and their cultures.
How do you design responsible AI?
AI models should be created with concrete goals that focus on building a model in a safe, trustworthy and ethical way. Ongoing scrutiny is crucial to ensure an organization is committed to providing unbiased, trustworthy AI technology. To do this, an organization must follow a maturity model while designing and implementing an AI system.
At a base level, responsible AI is built around development standards that focus on the principles for responsible design. These company-wide AI development standards should include the following mandates:
- Shared code repositories.
- Approved model architectures.
- Sanctioned variables.
- Established bias testing methodologies to help determine the validity of tests for AI systems.
- Stability standards for active machine learning models to ensure AI programming works as intended.
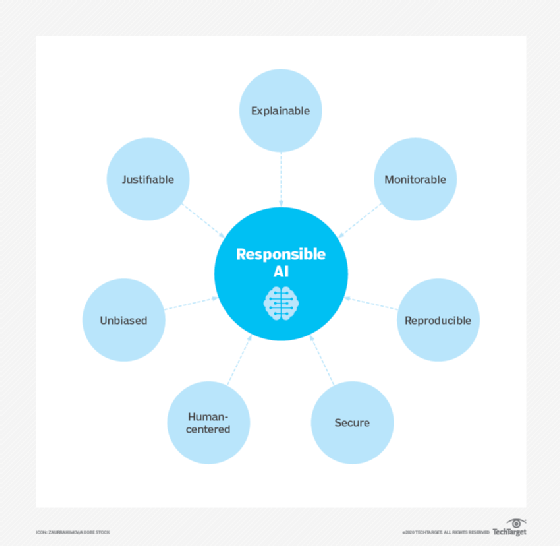
Responsible AI implementation and how it works
An organization can implement responsible AI and demonstrate that it has created a responsible AI system in the following ways:
- Use interpretable features to create data people can understand.
- Document design and decision-making processes so that if a mistake occurs, it can be reverse-engineered to determine what transpired.
- Build a diverse work culture and promote constructive discussions to help mitigate bias.
- Create a rigorous development process that values visibility into each application's latent features.
- Eliminate black box AI model development methods. Instead, use white box or explainable AI methods that provide an explanation for each decision the AI makes.
What are the key challenges in implementing responsible AI?
Responsible AI policies and frameworks aren't always easy to implement, because key challenges and concerns often slow down the process. These challenges include the following:
- Security and privacy. Businesses collecting data for AI model training might require confidential data on individuals. Responsible AI guidelines aim to address data privacy, data protection and security, yet it's often difficult to separate sensitive data from public data.
- Data bias. Training data should be properly sourced and scrutinized to avoid biases, but biases are often hard to detect. There's no perfect approach; eliminating biases in data sets and inputs requires time and effort.
- Compliance. As laws and regulations continue to evolve at the local, state, national and international levels, businesses must monitor new rules and ensure their AI policies are easy to update.
- Training. Business leaders must know who's involved in overseeing AI systems and ensure they're trained. In addition to technology teams, legal, marketing, human resources and other departments and stakeholders might need training.
Best practices for responsible AI principles
When designing responsible AI, development and deployment processes need to be systematic and repeatable. Some best practices include the following:
- Implement machine learning best practices.
- Create a diverse culture of support. This includes creating gender and racially diverse teams that work on creating responsible AI standards. Enable this culture to speak freely on ethical concepts around AI and bias.
- Promote transparency to create an explainable AI model so that any decisions it makes are visible and easy to fix if there's a problem.
- Make the work as measurable as possible. Dealing with responsibility is subjective, so ensure there are measurable processes in place such as visibility and explainability, and that there are auditable technical frameworks and ethical frameworks.
- Use responsible AI tools to inspect the models.
- Identify metrics for training and monitoring to help keep errors, false positives and biases at a minimum.
- Document best practices and benchmark them against one or more existing AI governance frameworks. Once these approaches are found to meet the principles of existing frameworks, this documentation can serve as an effective AI governance framework for an organization.
- Perform tests such as bias testing and predictive maintenance to help produce verifiable results and increase end-user trust.
- Monitor after deployment. This ensures the AI model continues to function in a responsible, unbiased way.
- Stay mindful and learn from the process. An organization discovers more about responsible AI in implementation, from fairness practices to technical references and materials surrounding technical ethics.
Best practices for responsible AI governance
From an oversight perspective, organizations should have an AI governance policy that's reusable for each AI system they develop or implement. Governance policies for responsible AI should include the following best practices:
- Transparency. Organizations should clearly state how they're using AI to develop, deploy and maintain algorithms, products and services.
- Accountability. A governance structure should be in place to provide effective monitoring and oversight.
- Ethical data use. AI teams must understand the implications of training an AI model on sensitive data and how to prevent biased or poisoned data. A governance policy keeps the concept of responsible AI top of mind for these developers, ensuring they closely examine data sources.
- Compliance. A policy must direct legal and compliance teams to work with AI developers to ensure development is in line with local, state and federal laws and regulations.
- Training. Each manager and employee involved with AI development, deployment and use should undergo training in what the governance policy is and how it works.
- Involvement of multiple teams. Depending on the needs of the enterprise, multiple teams might be involved in developing, implementing and maintaining a new AI product or tool. For example, when an AI model is developed to handle personal health information, teams of healthcare experts will likely work alongside developers.
Examples of companies embracing responsible AI
Among the companies pursuing responsible AI strategies and use cases are Microsoft, FICO and IBM.
Microsoft
Microsoft has created a responsible AI governance framework with help from its AI, Ethics and Effects in Engineering and Research Committee and Office of Responsible AI (ORA) groups. These two groups work together within Microsoft to spread and uphold responsible AI values.
ORA is responsible for setting company-wide rules for responsible AI through the implementation of governance and public policy work. Microsoft has implemented several responsible AI guidelines, checklists and templates, including the following:
- Human-AI interaction guidelines.
- Conversational AI guidelines.
- Inclusive design guidelines.
- AI fairness checklists.
- Templates for data sheets.
- AI security engineering guidance.
FICO
Credit scoring organization FICO has created responsible AI governance policies to help its employees and customers understand how the ML models the company uses work and their limitations. FICO's data scientists are tasked with considering the entire lifecycle of its ML models. They're constantly testing their effectiveness and fairness. FICO has developed the following methodologies and initiatives for bias detection:
- Build, execute and monitor explainable models for AI.
- Use blockchain as a governance tool for documenting how an AI solution works.
- Share an explainable AI toolkit with employees and clients.
- Use comprehensive testing for bias.
IBM
IBM has its own ethics board dedicated to issues surrounding artificial intelligence. The IBM AI Ethics Board is a central body that supports the creation of responsible and ethical AI throughout the company. Guidelines and resources IBM uses include the following:
- AI trust and transparency.
- Everyday ethical considerations for AI.
- Open source community resources.
- Research into trusted AI.
Blockchain's role in responsible AI
Blockchain is a popular distributed ledger technology used for tracking cryptocurrency transactional data. It's also a valuable tool for creating a tamper-proof record that documents why an ML model made a particular prediction. That's why some companies are using blockchain technology to document their AI use.
With blockchain, each step in the development process -- including who made, tested and approved each decision -- is recorded in a human-readable format that can't be altered.
Responsible AI standardization
Top executives at large companies, such as IBM, have publicly called for AI regulations. In the U.S., no federal laws or standards have yet emerged, even with the recent boom in generative AI models, such as ChatGPT. However, the EU AI Act of 2024 provides a framework to root out high-risk AI systems and protect sensitive data from misuse by such systems.
The U.S. has yet to pass federal legislation governing AI, and there are conflicting opinions on whether AI regulation is on the horizon. However, both NIST and the Biden administration have published broad guidelines for the use of AI. NIST has issued its Artificial Intelligence Risk Management Framework. The Biden administration has published blueprints for an AI Bill of Rights, an AI Risk Management Framework and a roadmap for creating a National AI Research Resource.
In March 2024, the European Parliament ratified the EU AI Act, which includes a regulatory framework for responsible AI practices. It takes a risk-based approach, dividing AI applications into four different categories: minimal risk, limited risk, high risk and unacceptable risk. High and unacceptable risk AI systems require mitigation over time. This law applies to both EU and non-EU that handle EU citizens' data.
The EU AI Act has clear implications for how businesses implement and use AI in the real world. Learn what you need to know about complying with the EU AI Act.