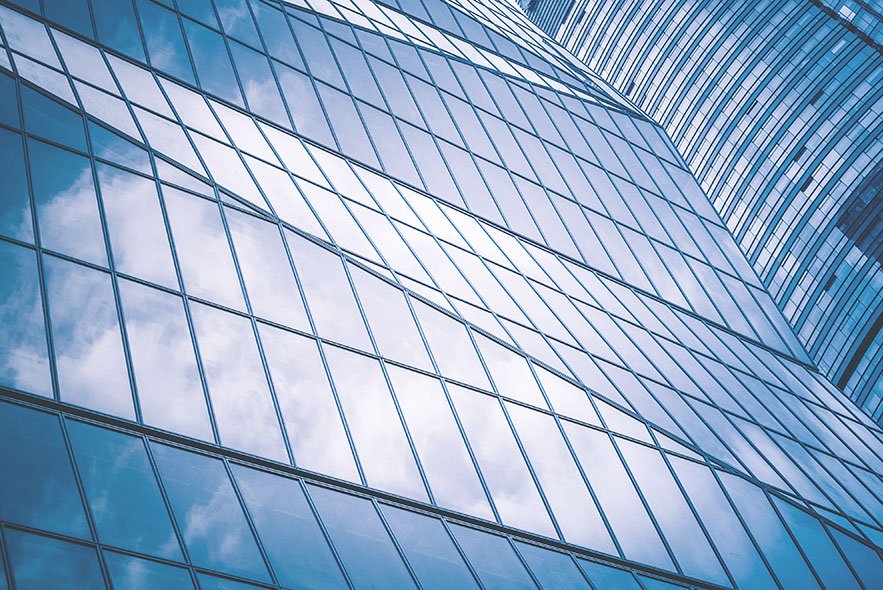
What is artificial intelligence (AI)? Everything you need to know
What is AI?
Artificial intelligence is the simulation of human intelligence processes by machines, especially computer systems. Specific applications of AI include expert systems, natural language processing, speech recognition and machine vision.
How does AI work?
As the hype around AI has accelerated, vendors have been scrambling to promote how their products and services use it. Often, what they refer to as AI is simply a component of the technology, such as machine learning. AI requires a foundation of specialized hardware and software for writing and training machine learning algorithms. No single programming language is synonymous with AI, but Python, R, Java, C++ and Julia have features popular with AI developers.
In general, AI systems work by ingesting large amounts of labeled training data, analyzing the data for correlations and patterns, and using these patterns to make predictions about future states. In this way, a chatbot that is fed examples of text can learn to generate lifelike exchanges with people, or an image recognition tool can learn to identify and describe objects in images by reviewing millions of examples. New, rapidly improving generative AI techniques can create realistic text, images, music and other media.
AI programming focuses on cognitive skills that include the following:
- Learning. This aspect of AI programming focuses on acquiring data and creating rules for how to turn it into actionable information. The rules, which are called algorithms, provide computing devices with step-by-step instructions for how to complete a specific task.
- Reasoning. This aspect of AI programming focuses on choosing the right algorithm to reach a desired outcome.
- Self-correction. This aspect of AI programming is designed to continually fine-tune algorithms and ensure they provide the most accurate results possible.
- Creativity. This aspect of AI uses neural networks, rules-based systems, statistical methods and other AI techniques to generate new images, new text, new music and new ideas.
Differences between AI, machine learning and deep learning
AI, machine learning and deep learning are common terms in enterprise IT and sometimes used interchangeably, especially by companies in their marketing materials. But there are distinctions. The term AI, coined in the 1950s, refers to the simulation of human intelligence by machines. It covers an ever-changing set of capabilities as new technologies are developed. Technologies that come under the umbrella of AI include machine learning and deep learning.
Machine learning enables software applications to become more accurate at predicting outcomes without being explicitly programmed to do so. Machine learning algorithms use historical data as input to predict new output values. This approach became vastly more effective with the rise of large data sets to train on. Deep learning, a subset of machine learning, is based on our understanding of how the brain is structured. Deep learning's use of artificial neural network structure is the underpinning of recent advances in AI, including self-driving cars and ChatGPT.
Why is artificial intelligence important?
AI is important for its potential to change how we live, work and play. It has been effectively used in business to automate tasks done by humans, including customer service work, lead generation, fraud detection and quality control. In a number of areas, AI can perform tasks much better than humans. Particularly when it comes to repetitive, detail-oriented tasks, such as analyzing large numbers of legal documents to ensure relevant fields are filled in properly, AI tools often complete jobs quickly and with relatively few errors. Because of the massive data sets it can process, AI can also give enterprises insights into their operations they might not have been aware of. The rapidly expanding population of generative AI tools will be important in fields ranging from education and marketing to product design.
This article is part of
A guide to artificial intelligence in the enterprise
Indeed, advances in AI techniques have not only helped fuel an explosion in efficiency, but opened the door to entirely new business opportunities for some larger enterprises. Prior to the current wave of AI, it would have been hard to imagine using computer software to connect riders to taxis, but Uber has become a Fortune 500 company by doing just that.
AI has become central to many of today's largest and most successful companies, including Alphabet, Apple, Microsoft and Meta, where AI technologies are used to improve operations and outpace competitors. At Alphabet subsidiary Google, for example, AI is central to its search engine, Waymo's self-driving cars and Google Brain, which invented the transformer neural network architecture that underpins the recent breakthroughs in natural language processing.
What are the advantages and disadvantages of artificial intelligence?
Artificial neural networks and deep learning AI technologies are quickly evolving, primarily because AI can process large amounts of data much faster and make predictions more accurately than humanly possible.
While the huge volume of data created on a daily basis would bury a human researcher, AI applications using machine learning can take that data and quickly turn it into actionable information. As of this writing, a primary disadvantage of AI is that it is expensive to process the large amounts of data AI programming requires. As AI techniques are incorporated into more products and services, organizations must also be attuned to AI's potential to create biased and discriminatory systems, intentionally or inadvertently.
Advantages of AI
The following are some advantages of AI.
- Good at detail-oriented jobs. AI has proven to be just as good, if not better than doctors at diagnosing certain cancers, including breast cancer and melanoma.
- Reduced time for data-heavy tasks. AI is widely used in data-heavy industries, including banking and securities, pharma and insurance, to reduce the time it takes to analyze big data sets. Financial services, for example, routinely use AI to process loan applications and detect fraud.
- Saves labor and increases productivity. An example here is the use of warehouse automation, which grew during the pandemic and is expected to increase with the integration of AI and machine learning.
- Delivers consistent results. The best AI translation tools deliver high levels of consistency, offering even small businesses the ability to reach customers in their native language.
- Can improve customer satisfaction through personalization. AI can personalize content, messaging, ads, recommendations and websites to individual customers.
- AI-powered virtual agents are always available. AI programs do not need to sleep or take breaks, providing 24/7 service.
Disadvantages of AI
The following are some disadvantages of AI.
- Expensive.
- Requires deep technical expertise.
- Limited supply of qualified workers to build AI tools.
- Reflects the biases of its training data, at scale.
- Lack of ability to generalize from one task to another.
- Eliminates human jobs, increasing unemployment rates.
Strong AI vs. weak AI
AI can be categorized as weak or strong.
- Weak AI, also known as narrow AI, is designed and trained to complete a specific task. Industrial robots and virtual personal assistants, such as Apple's Siri, use weak AI.
- Strong AI, also known as artificial general intelligence (AGI), describes programming that can replicate the cognitive abilities of the human brain. When presented with an unfamiliar task, a strong AI system can use fuzzy logic to apply knowledge from one domain to another and find a solution autonomously. In theory, a strong AI program should be able to pass both a Turing test and the Chinese Room argument.
What are the 4 types of artificial intelligence?
Arend Hintze, an assistant professor of integrative biology and computer science and engineering at Michigan State University, explained that AI can be categorized into four types, beginning with the task-specific intelligent systems in wide use today and progressing to sentient systems, which do not yet exist. The categories are as follows.
- Type 1: Reactive machines. These AI systems have no memory and are task-specific. An example is Deep Blue, the IBM chess program that beat Garry Kasparov in the 1990s. Deep Blue can identify pieces on a chessboard and make predictions, but because it has no memory, it cannot use past experiences to inform future ones.
- Type 2: Limited memory. These AI systems have memory, so they can use past experiences to inform future decisions. Some of the decision-making functions in self-driving cars are designed this way.
- Type 3: Theory of mind. Theory of mind is a psychology term. When applied to AI, it means the system would have the social intelligence to understand emotions. This type of AI will be able to infer human intentions and predict behavior, a necessary skill for AI systems to become integral members of human teams.
- Type 4: Self-awareness. In this category, AI systems have a sense of self, which gives them consciousness. Machines with self-awareness understand their own current state. This type of AI does not yet exist.

What are examples of AI technology and how is it used today?
AI is incorporated into a variety of different types of technology. Here are seven examples.
Automation. When paired with AI technologies, automation tools can expand the volume and types of tasks performed. An example is robotic process automation (RPA), a type of software that automates repetitive, rules-based data processing tasks traditionally done by humans. When combined with machine learning and emerging AI tools, RPA can automate bigger portions of enterprise jobs, enabling RPA's tactical bots to pass along intelligence from AI and respond to process changes.
Machine learning. This is the science of getting a computer to act without programming. Deep learning is a subset of machine learning that, in very simple terms, can be thought of as the automation of predictive analytics. There are three types of machine learning algorithms:
- Supervised learning. Data sets are labeled so that patterns can be detected and used to label new data sets.
- Unsupervised learning. Data sets aren't labeled and are sorted according to similarities or differences.
- Reinforcement learning. Data sets aren't labeled but, after performing an action or several actions, the AI system is given feedback.
Machine vision. This technology gives a machine the ability to see. Machine vision captures and analyzes visual information using a camera, analog-to-digital conversion and digital signal processing. It is often compared to human eyesight, but machine vision isn't bound by biology and can be programmed to see through walls, for example. It is used in a range of applications from signature identification to medical image analysis. Computer vision, which is focused on machine-based image processing, is often conflated with machine vision.
Natural language processing (NLP). This is the processing of human language by a computer program. One of the older and best-known examples of NLP is spam detection, which looks at the subject line and text of an email and decides if it's junk. Current approaches to NLP are based on machine learning. NLP tasks include text translation, sentiment analysis and speech recognition.
Robotics. This field of engineering focuses on the design and manufacturing of robots. Robots are often used to perform tasks that are difficult for humans to perform or perform consistently. For example, robots are used in car production assembly lines or by NASA to move large objects in space. Researchers also use machine learning to build robots that can interact in social settings.
Self-driving cars. Autonomous vehicles use a combination of computer vision, image recognition and deep learning to build automated skills to pilot a vehicle while staying in a given lane and avoiding unexpected obstructions, such as pedestrians.
Text, image and audio generation. Generative AI techniques, which create various types of media from text prompts, are being applied extensively across businesses to create a seemingly limitless range of content types from photorealistic art to email responses and screenplays.

What are the applications of AI?
Artificial intelligence has made its way into a wide variety of markets. Here are 11 examples.
AI in healthcare. The biggest bets are on improving patient outcomes and reducing costs. Companies are applying machine learning to make better and faster medical diagnoses than humans. One of the best-known healthcare technologies is IBM Watson. It understands natural language and can respond to questions asked of it. The system mines patient data and other available data sources to form a hypothesis, which it then presents with a confidence scoring schema. Other AI applications include using online virtual health assistants and chatbots to help patients and healthcare customers find medical information, schedule appointments, understand the billing process and complete other administrative processes. An array of AI technologies is also being used to predict, fight and understand pandemics such as COVID-19.
AI in business. Machine learning algorithms are being integrated into analytics and customer relationship management (CRM) platforms to uncover information on how to better serve customers. Chatbots have been incorporated into websites to provide immediate service to customers. The rapid advancement of generative AI technology such as ChatGPT is expected to have far-reaching consequences: eliminating jobs, revolutionizing product design and disrupting business models.
AI in education. AI can automate grading, giving educators more time for other tasks. It can assess students and adapt to their needs, helping them work at their own pace. AI tutors can provide additional support to students, ensuring they stay on track. The technology could also change where and how students learn, perhaps even replacing some teachers. As demonstrated by ChatGPT, Google Bard and other large language models, generative AI can help educators craft course work and other teaching materials and engage students in new ways. The advent of these tools also forces educators to rethink student homework and testing and revise policies on plagiarism.
AI in finance. AI in personal finance applications, such as Intuit Mint or TurboTax, is disrupting financial institutions. Applications such as these collect personal data and provide financial advice. Other programs, such as IBM Watson, have been applied to the process of buying a home. Today, artificial intelligence software performs much of the trading on Wall Street.
AI in law. The discovery process -- sifting through documents -- in law is often overwhelming for humans. Using AI to help automate the legal industry's labor-intensive processes is saving time and improving client service. Law firms use machine learning to describe data and predict outcomes, computer vision to classify and extract information from documents, and NLP to interpret requests for information.
AI in entertainment and media. The entertainment business uses AI techniques for targeted advertising, recommending content, distribution, detecting fraud, creating scripts and making movies. Automated journalism helps newsrooms streamline media workflows reducing time, costs and complexity. Newsrooms use AI to automate routine tasks, such as data entry and proofreading; and to research topics and assist with headlines. How journalism can reliably use ChatGPT and other generative AI to generate content is open to question.
AI in software coding and IT processes. New generative AI tools can be used to produce application code based on natural language prompts, but it is early days for these tools and unlikely they will replace software engineers soon. AI is also being used to automate many IT processes, including data entry, fraud detection, customer service, and predictive maintenance and security.
Security. AI and machine learning are at the top of the buzzword list security vendors use to market their products, so buyers should approach with caution. Still, AI techniques are being successfully applied to multiple aspects of cybersecurity, including anomaly detection, solving the false-positive problem and conducting behavioral threat analytics. Organizations use machine learning in security information and event management (SIEM) software and related areas to detect anomalies and identify suspicious activities that indicate threats. By analyzing data and using logic to identify similarities to known malicious code, AI can provide alerts to new and emerging attacks much sooner than human employees and previous technology iterations.
AI in manufacturing. Manufacturing has been at the forefront of incorporating robots into the workflow. For example, the industrial robots that were at one time programmed to perform single tasks and separated from human workers, increasingly function as cobots: Smaller, multitasking robots that collaborate with humans and take on responsibility for more parts of the job in warehouses, factory floors and other workspaces.
AI in banking. Banks are successfully employing chatbots to make their customers aware of services and offerings and to handle transactions that don't require human intervention. AI virtual assistants are used to improve and cut the costs of compliance with banking regulations. Banking organizations use AI to improve their decision-making for loans, set credit limits and identify investment opportunities.
AI in transportation. In addition to AI's fundamental role in operating autonomous vehicles, AI technologies are used in transportation to manage traffic, predict flight delays, and make ocean shipping safer and more efficient. In supply chains, AI is replacing traditional methods of forecasting demand and predicting disruptions, a trend accelerated by COVID-19 when many companies were caught off guard by the effects of a global pandemic on the supply and demand of goods.
Augmented intelligence vs. artificial intelligence
Some industry experts have argued that the term artificial intelligence is too closely linked to popular culture, which has caused the general public to have improbable expectations about how AI will change the workplace and life in general. They have suggested using the term augmented intelligence to differentiate between AI systems that act autonomously -- popular culture examples include Hal 9000 and The Terminator -- and AI tools that support humans.
- Augmented intelligence. Some researchers and marketers hope the label augmented intelligence, which has a more neutral connotation, will help people understand that most implementations of AI will be weak and simply improve products and services. Examples include automatically surfacing important information in business intelligence reports or highlighting important information in legal filings. The rapid adoption of ChatGPT and Bard across industry indicates a willingness to use AI to support human decision-making.
- Artificial intelligence. True AI, or AGI, is closely associated with the concept of the technological singularity -- a future ruled by an artificial superintelligence that far surpasses the human brain's ability to understand it or how it is shaping our reality. This remains within the realm of science fiction, though some developers are working on the problem. Many believe that technologies such as quantum computing could play an important role in making AGI a reality and that we should reserve the use of the term AI for this kind of general intelligence.
Ethical use of artificial intelligence
While AI tools present a range of new functionality for businesses, the use of AI also raises ethical questions because, for better or worse, an AI system will reinforce what it has already learned.
This can be problematic because machine learning algorithms, which underpin many of the most advanced AI tools, are only as smart as the data they are given in training. Because a human being selects what data is used to train an AI program, the potential for machine learning bias is inherent and must be monitored closely.
Anyone looking to use machine learning as part of real-world, in-production systems needs to factor ethics into their AI training processes and strive to avoid bias. This is especially true when using AI algorithms that are inherently unexplainable in deep learning and generative adversarial network (GAN) applications.
Explainability is a potential stumbling block to using AI in industries that operate under strict regulatory compliance requirements. For example, financial institutions in the United States operate under regulations that require them to explain their credit-issuing decisions. When a decision to refuse credit is made by AI programming, however, it can be difficult to explain how the decision was arrived at because the AI tools used to make such decisions operate by teasing out subtle correlations between thousands of variables. When the decision-making process cannot be explained, the program may be referred to as black box AI.
In summary, AI's ethical challenges include the following:
- Bias due to improperly trained algorithms and human bias.
- Misuse due to deepfakes and phishing.
- Legal concerns, including AI libel and copyright issues.
- Elimination of jobs due to the growing capabilities of AI.
- Data privacy concerns, particularly in the banking, healthcare and legal fields.
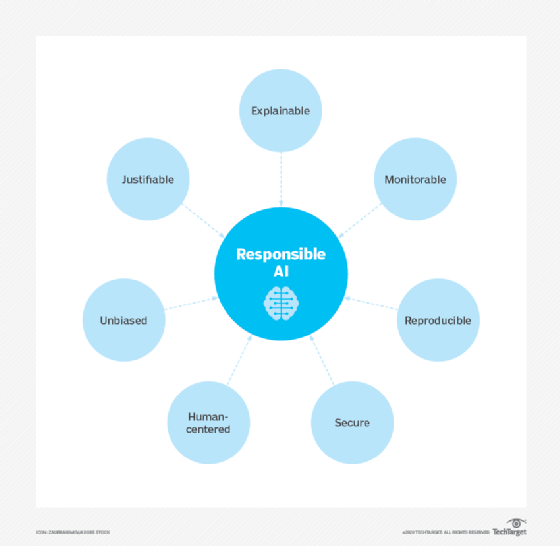
AI governance and regulations
Despite potential risks, there are currently few regulations governing the use of AI tools, and where laws do exist, they typically pertain to AI indirectly. For example, as previously mentioned, U.S. Fair Lending regulations require financial institutions to explain credit decisions to potential customers. This limits the extent to which lenders can use deep learning algorithms, which by their nature are opaque and lack explainability.
The European Union's General Data Protection Regulation (GDPR) is considering AI regulations. GDPR's strict limits on how enterprises can use consumer data already limits the training and functionality of many consumer-facing AI applications.
Policymakers in the U.S. have yet to issue AI legislation, but that could change soon. A "Blueprint for an AI Bill of Rights" published in October 2022 by the White House Office of Science and Technology Policy (OSTP) guides businesses on how to implement ethical AI systems. The U.S. Chamber of Commerce also called for AI regulations in a report released in March 2023.
Crafting laws to regulate AI will not be easy, in part because AI comprises a variety of technologies that companies use for different ends, and partly because regulations can come at the cost of AI progress and development. The rapid evolution of AI technologies is another obstacle to forming meaningful regulation of AI, as are the challenges presented by AI's lack of transparency that make it difficult to see how the algorithms reach their results. Moreover, technology breakthroughs and novel applications such as ChatGPT and Dall-E can make existing laws instantly obsolete. And, of course, the laws that governments do manage to craft to regulate AI don't stop criminals from using the technology with malicious intent.
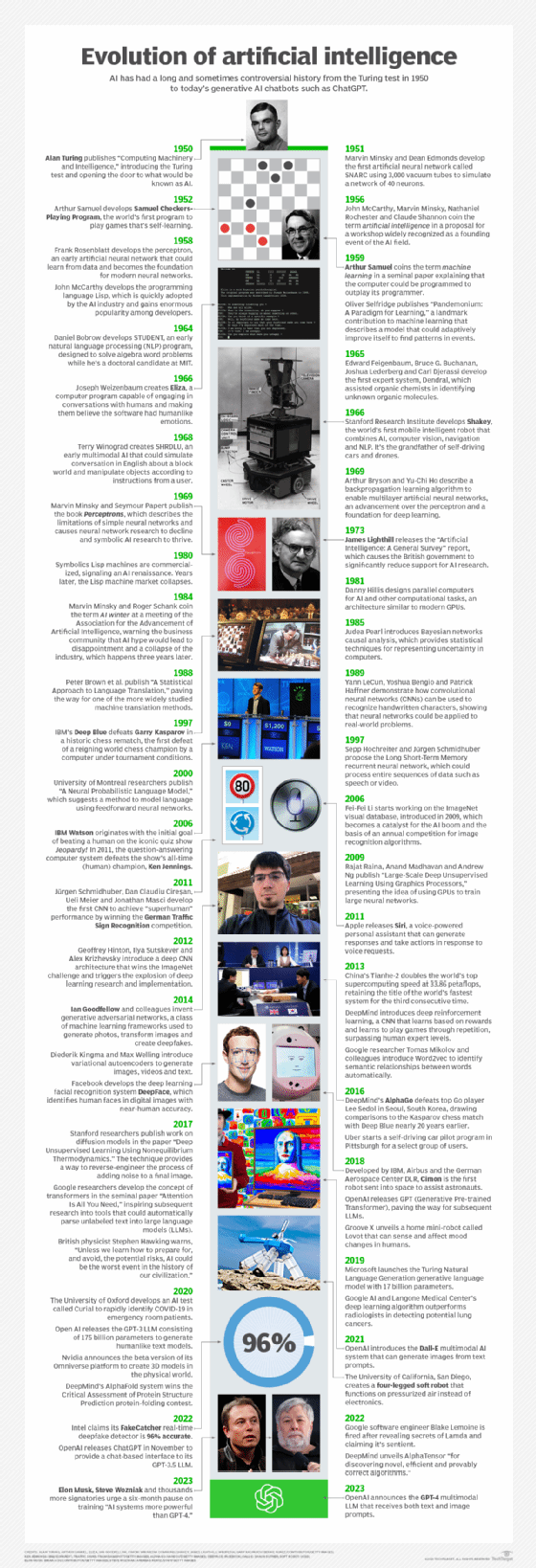
What is the history of AI?
The concept of inanimate objects endowed with intelligence has been around since ancient times. The Greek god Hephaestus was depicted in myths as forging robot-like servants out of gold. Engineers in ancient Egypt built statues of gods animated by priests. Throughout the centuries, thinkers from Aristotle to the 13th century Spanish theologian Ramon Llull to René Descartes and Thomas Bayes used the tools and logic of their times to describe human thought processes as symbols, laying the foundation for AI concepts such as general knowledge representation.
The late 19th and first half of the 20th centuries brought forth the foundational work that would give rise to the modern computer. In 1836, Cambridge University mathematician Charles Babbage and Augusta Ada King, Countess of Lovelace, invented the first design for a programmable machine.
1940s. Princeton mathematician John Von Neumann conceived the architecture for the stored-program computer -- the idea that a computer's program and the data it processes can be kept in the computer's memory. And Warren McCulloch and Walter Pitts laid the foundation for neural networks.
1950s. With the advent of modern computers, scientists could test their ideas about machine intelligence. One method for determining whether a computer has intelligence was devised by the British mathematician and World War II code-breaker Alan Turing. The Turing test focused on a computer's ability to fool interrogators into believing its responses to their questions were made by a human being.
1956. The modern field of artificial intelligence is widely cited as starting this year during a summer conference at Dartmouth College. Sponsored by the Defense Advanced Research Projects Agency (DARPA), the conference was attended by 10 luminaries in the field, including AI pioneers Marvin Minsky, Oliver Selfridge and John McCarthy, who is credited with coining the term artificial intelligence. Also in attendance were Allen Newell, a computer scientist, and Herbert A. Simon, an economist, political scientist and cognitive psychologist. The two presented their groundbreaking Logic Theorist, a computer program capable of proving certain mathematical theorems and referred to as the first AI program.
1950s and 1960s. In the wake of the Dartmouth College conference, leaders in the fledgling field of AI predicted that a man-made intelligence equivalent to the human brain was around the corner, attracting major government and industry support. Indeed, nearly 20 years of well-funded basic research generated significant advances in AI: For example, in the late 1950s, Newell and Simon published the General Problem Solver (GPS) algorithm, which fell short of solving complex problems but laid the foundations for developing more sophisticated cognitive architectures; and McCarthy developed Lisp, a language for AI programming still used today. In the mid-1960s, MIT Professor Joseph Weizenbaum developed ELIZA, an early NLP program that laid the foundation for today's chatbots.
1970s and 1980s. The achievement of artificial general intelligence proved elusive, not imminent, hampered by limitations in computer processing and memory and by the complexity of the problem. Government and corporations backed away from their support of AI research, leading to a fallow period lasting from 1974 to 1980 known as the first "AI Winter." In the 1980s, research on deep learning techniques and industry's adoption of Edward Feigenbaum's expert systems sparked a new wave of AI enthusiasm, only to be followed by another collapse of government funding and industry support. The second AI winter lasted until the mid-1990s.
1990s. Increases in computational power and an explosion of data sparked an AI renaissance in the late 1990s that set the stage for the remarkable advances in AI we see today. The combination of big data and increased computational power propelled breakthroughs in NLP, computer vision, robotics, machine learning and deep learning. In 1997, as advances in AI accelerated, IBM's Deep Blue defeated Russian chess grandmaster Garry Kasparov, becoming the first computer program to beat a world chess champion.
2000s. Further advances in machine learning, deep learning, NLP, speech recognition and computer vision gave rise to products and services that have shaped the way we live today. These include the 2000 launch of Google's search engine and the 2001 launch of Amazon's recommendation engine. Netflix developed its recommendation system for movies, Facebook introduced its facial recognition system and Microsoft launched its speech recognition system for transcribing speech into text. IBM launched Watson and Google started its self-driving initiative, Waymo.
2010s. The decade between 2010 and 2020 saw a steady stream of AI developments. These include the launch of Apple's Siri and Amazon's Alexa voice assistants; IBM Watson's victories on Jeopardy; self-driving cars; the development of the first generative adversarial network; the launch of TensorFlow, Google's open source deep learning framework; the founding of research lab OpenAI, developers of the GPT-3 language model and Dall-E image generator; the defeat of world Go champion Lee Sedol by Google DeepMind's AlphaGo; and the implementation of AI-based systems that detect cancers with a high degree of accuracy.
2020s. The current decade has seen the advent of generative AI, a type of artificial intelligence technology that can produce new content. Generative AI starts with a prompt that could be in the form of a text, an image, a video, a design, musical notes or any input that the AI system can process. Various AI algorithms then return new content in response to the prompt. Content can include essays, solutions to problems, or realistic fakes created from pictures or audio of a person. The abilities of language models such as ChatGPT-3, Google's Bard and Microsoft's Megatron-Turing NLG have wowed the world, but the technology is still in early stages, as evidenced by its tendency to hallucinate or skew answers.
AI tools and services
AI tools and services are evolving at a rapid rate. Current innovations in AI tools and services can be traced to the 2012 AlexNet neural network that ushered in a new era of high-performance AI built on GPUs and large data sets. The key change was the ability to train neural networks on massive amounts of data across multiple GPU cores in parallel in a more scalable way.
Over the last several years, the symbiotic relationship between AI discoveries at Google, Microsoft, and OpenAI, and the hardware innovations pioneered by Nvidia have enabled running ever-larger AI models on more connected GPUs, driving game-changing improvements in performance and scalability.
The collaboration among these AI luminaries was crucial for the recent success of ChatGPT, not to mention dozens of other breakout AI services. Here is a rundown of important innovations in AI tools and services.
Transformers. Google, for example, led the way in finding a more efficient process for provisioning AI training across a large cluster of commodity PCs with GPUs. This paved the way for the discovery of transformers that automate many aspects of training AI on unlabeled data.
Hardware optimization. Just as important, hardware vendors like Nvidia are also optimizing the microcode for running across multiple GPU cores in parallel for the most popular algorithms. Nvidia claimed the combination of faster hardware, more efficient AI algorithms, fine-tuning GPU instructions and better data center integration is driving a million-fold improvement in AI performance. Nvidia is also working with all cloud center providers to make this capability more accessible as AI-as-a-Service through IaaS, SaaS and PaaS models.
Generative pre-trained transformers. The AI stack has also evolved rapidly over the last few years. Previously enterprises would have to train their AI models from scratch. Increasingly vendors such as OpenAI, Nvidia, Microsoft, Google, and others provide generative pre-trained transformers (GPTs), which can be fine-tuned for a specific task at a dramatically reduced cost, expertise and time. Whereas some of the largest models are estimated to cost $5 million to $10 million per run, enterprises can fine-tune the resulting models for a few thousand dollars. This results in faster time to market and reduces risk.
AI cloud services. Among the biggest roadblocks that prevent enterprises from effectively using AI in their businesses are the data engineering and data science tasks required to weave AI capabilities into new apps or to develop new ones. All the leading cloud providers are rolling out their own branded AI as service offerings to streamline data prep, model development and application deployment. Top examples include AWS AI Services, Google Cloud AI, Microsoft Azure AI platform, IBM AI solutions and Oracle Cloud Infrastructure AI Services.
Cutting-edge AI models as a service. Leading AI model developers also offer cutting-edge AI models on top of these cloud services. OpenAI has dozens of large language models optimized for chat, NLP, image generation and code generation that are provisioned through Azure. Nvidia has pursued a more cloud-agnostic approach by selling AI infrastructure and foundational models optimized for text, images and medical data available across all cloud providers. Hundreds of other players are offering models customized for various industries and use cases as well.
George Lawton also contributed to this article.