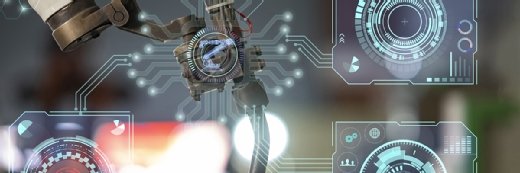
Getty Images/
Physical AI explained: Everything you need to know
AI has lived in the digital world, limited to algorithms and software. Now, with physical AI, robotics and AI merge, creating new frontiers and challenges for us to explore.
As AI continues to evolve, the focus is shifting toward improving machine-to-human AI interaction, giving rise to the field of physical AI.
Today, the most talked about form of AI is generative AI, which involves human-to-machine interaction. However, an effort is underway to improve the converse and make machine-to-human AI interaction more accessible and capable.
These machine-to-human interactions fall under the umbrella of physical AI, also known as physical agents or embodied AI, which employs AI techniques to solve problems that involve direct interaction between machines and the physical world. In addition, physical AI improves and expands its capabilities through its continued observations of and interactions with the physical world.
What is physical AI?
Physical AI creates systems that learn about and understand an environment directly from sensor data. Indeed, its primary input providers are sensors and actuators. Whereas generative AI requires human input, physical AI systems receive input from many tools, including cameras, microphones, temperature gauges, inertial sensors, radar and lidar.
Also, while the passage of time does not affect generative AI processes, physical AI systems require real-time perception and reasoning to understand an environment, and then react quickly and accordingly.
Characteristics of physical AI
Physical AI needs an actuator -- robotic arms, wheels or any other device -- that moves through or interacts with an environment and enables modification or manipulation of physical objects in that environment.
Physical AI systems have greater autonomy than those in generative AI. They don't require humans to initiate actions. They make decisions based on their perception of the environment and their programming. Since learning and adaptation go hand in hand with autonomy, many physical AI systems contain learning algorithms to recognize environmental changes, adapt as needed and improve their performance over time.
Currently, most physical AI systems are limited to specific tasks or small environments with mixed results at best. Commercially, a well-known example is the Roomba autonomous floor cleaner.
Physical AI and robotics
Physical AI's interactive systems are tightly interwoven with robotics, enabling AI-powered robots to perceive, reason and act autonomously in their environments.
Traditionally, robotics relied on a variety of input devices, such as cameras, lidar, sonar and other environmental sensors. The key difference between older robotic systems and those powered by physical AI is autonomy in decision-making. Older robots are preprogrammed to make decisions or react in a certain way to hard coding.
A physical AI-powered robot relies on real-time data amid changing environmental conditions to make its decision on the spot, then learn from those decisions and adapt to similar situations. As such, these robots rely on neural networks and deep learning to analyze their experiences and improve future reactions and interactions.
Physical AI robots employ advanced actuators -- driven by AI and machine learning (ML), far surpassing current models and designs -- to interact with their environment, moving through and manipulating objects in it.
What is unique about physical AI?
Physical AI interacts with the environment around it -- and not necessarily humans. Rather than asking a question like generative AI does, physical AI operates and adjusts within the physical world based on input from sensors and actuators.
Of course, when interacting with humans and operating autonomously, physical AI's safety and interaction protocols are paramount, ranging from human proximity alerts and collision avoidance to the ability to recognize facial expressions and even attempt to understand human intentions.
Physical AI also requires work from multiple experts in various fields, drawing from the best in robotics, computer vision, ML, control theory and mechanical engineering to develop a properly functioning system.
Finally, because of their constant interactive nature, physical AI's unique systems demand powerful processing capabilities to respond effectively during dynamic environmental changes, necessitating software algorithms and hardware that make decisions in milliseconds.
Why is physical AI important?
Physical AI augments human work. Computers, after all, don't tire or err, making them invaluable in a sensitive environment, such as a hospital. For instance, an AI robot ensures that a patient receives medication on time and regular monitoring of vital statistics.
Physical AI also aids in data gathering. Again, sensors and real-time analytics monitor, for example, a sensitive manufacturing process. Furthermore, physical AI is essential in any environment where constant observation, alert notification and rapid response to troublesome data are the norm.
Taken to its extreme, physical AI is useful in hazardous environments or situations where human safety is a concern. Yes, current fire departments send robots to examine areas dangerous to humans, and bomb squads deploy robots capable of containing suspected explosives, avoiding danger to human life and limb. However, physical AI adds greater intelligence and learning ability to these machines, and it potentially eliminates human participation in a dangerous task.
The future of physical AI
At the beginning of the physical AI revolution, there is vast room for its application, growth and improvement. Built on considerable and continuous software and hardware development, physical AI promises to augment human activities and automate tasks that prove difficult, if not impossible, for its makers. Below are listed an array of fields tapped to benefit from physical AI in the future:
- Robotics and automation. Continuous advancements make robots more dexterous and agile with increased efficiency and precision in performing complex tasks. One particular area of application is robotics in the operating room, where a robot assists a physician with delicate surgery.
- Autonomous vehicles. There are already self-driving cars on the road. Results are mixed, and confidence in self-driving remains low. As sensor technology improves and neural networks train their systems, expect safer, more efficient road networks with an increasing number of autonomous vehicles, including trucks and drones.
- Human-robot collaboration. The example above of a surgical robot is just one instance of physical AI complementing and supplementing human activities. Anticipate greater human application of physical AI in tasks where other humans are either unavailable, placed in too much danger or unable to perform a task, such as one requiring tremendous strength.
- Environmental monitoring. As noted earlier, robots don't require rest. Autonomous drones and robots powered by physical AI monitor an environment -- from factory floors to farms -- around the clock. They can spot disasters, toxic buildup, fires, water level changes and other resource variations.
- AI-driven manufacturing. Manufacturing already features robotics, but advanced systems with physical AI deliver greater optimization of the production process, enhanced quality control, more flexible and versatile manufacturing systems and just-in-time manufacturing support.
- Technological expansion. Physical AI is currently isolated from other technologies such as 5G networks, edge computing, augmented reality and the internet of things. The addition of physical AI to remote connectivity enables new technological capabilities, real-time data processing, enhanced connectivity and improved decision-making capabilities for remote systems.
Andy Patrizio is a technology journalist with almost 30 years of experience covering Silicon Valley who has worked for a variety of publications -- on staff or as a freelancer -- including Network World, InfoWorld, Business Insider, Ars Technica and InformationWeek. He is currently based in southern California.