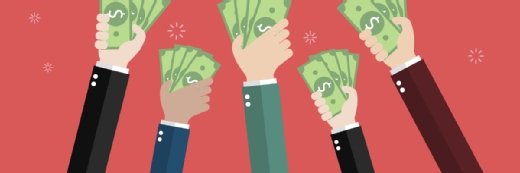
Getty Images
Personalized pricing explained: Everything you need to know
Personalized pricing is a strategy that sets different prices for the same product based on a customer's purchase history, location and browsing behavior.
Personalized pricing, a business strategy that adjusts prices based on individual customer characteristics, has recently come under increased scrutiny from regulatory bodies.
The Federal Trade Commission (FTC) recently launched an investigation on how companies, including McKinsey, Mastercard and Chase, use consumer data to construct personalized pricing strategies, also known as surveillance pricing or dynamic pricing strategies. The FTC's investigation reflects growing concerns about the transparency and fairness of personalized pricing as it becomes more prevalent in online retail and e-commerce.
The commission's inquiry questions whether these pricing models exploit personal data to the detriment of consumers, potentially leading to discriminatory practices. While proponents argue personalized pricing offers lower prices to consumers more sensitive to cost, critics worry it leads to higher prices for less price-sensitive customers, undermining overall consumer welfare and confidence.
Personalized pricing exists across industries. For instance, online travel agencies offer different prices for the same flight based on a user's search history or device type. Similarly, streaming services offer discounted subscription rates to new users while charging existing users higher renewal rates based on their loyalty and usage patterns. These practices are legally complex and raise questions about consumer data privacy and the ethical implications of price discrimination in a digital economy.
What is personalized pricing?
Personalized pricing is a strategy of setting prices for products or services based on individual customer data, rather than offering a fixed price to all consumers. This model tailors prices by analyzing a specific customer's purchase history, browsing behavior, location and demographic information. By using algorithms and big data analytics, businesses adjust prices to match what they believe an individual customer will pay.
Personalized pricing is rooted in price discrimination, a well-established principle in which sellers charge different prices to various consumers based on their willingness to pay. However, personalized pricing goes further by employing advanced technologies such as AI and machine learning (ML) to adjust prices dynamically at the individual level.
While personalized pricing offers targeted deals to enhance customer experiences, it raises concerns about fairness and transparency. Critics argue that, without proper regulation, personalized pricing unfairly disadvantages less tech-savvy consumers with limited access to competitive pricing information.
How does personalized pricing work?
Personalized pricing analyzes individual customer data to set tailored prices in real time. This process begins with collecting data points such as browsing history, purchase behavior and location, referencing them against existing demand. Advanced algorithms then segment customers based on their likelihood to pay a certain price, adjusting offers accordingly.
For example, a customer who frequently buys premium products typically sees higher prices, while a price-sensitive shopper regularly receives discounts. AI and ML continuously refine these algorithms, ensuring pricing strategies remain accurate and responsive to changing behaviors and market conditions.
Data-driven personalized pricing is common in e-commerce, online travel and subscription services, simultaneously increasing business revenue and customer engagement when done properly. Of course, its success depends on the quality of the data and sophistication of the algorithms.
How can AI be used in personalized pricing?
AI is a driving force behind effective personalized pricing strategies, enhancing the analysis of large quantities of customer data quickly and accurately and enabling businesses to set individualized prices in real time. Using machine learning algorithms, AI identifies patterns in consumer behavior, such as purchasing habits, browsing activity and even responses to previous pricing offers.
Indeed, AI's role in personalized pricing cannot be overstated. First, AI-powered models predict customer behavior by analyzing historical data and current interactions. These predictions enable businesses to estimate a customer's willingness to pay and adjust their prices accordingly. For instance, AI detects if a customer is more likely to wait to purchase during a sale or pay a premium for immediate availability.
Moreover, AI facilitates dynamic pricing by continuously monitoring external factors such as competitor prices, market demand and inventory levels. This allows businesses to adjust prices in real time, remaining competitive while maximizing revenue.
AI also personalizes the customer experience by delivering tailored offers and discounts. AI identifies which pricing tactics work best for various customer segments and continuously improves these strategies through A/B testing and other methods.
Further, AI's ability to process and learn continuously from data makes personalized pricing models more accurate and effective over time. As more customer interactions are analyzed, the algorithms improve, better-aligning pricing strategies with individual customer preferences and market conditions.
Examples of personalized pricing
Here are some everyday examples of personalized pricing strategies:
- Tailored pricing in online travel agencies. Online travel agencies adjust hotel or car rental prices for returning customers based on their previous booking patterns. A customer who regularly books high-end hotels sees higher-priced or premium options, while a price-sensitive traveler receives offers for budget-friendly accommodations or targeted discounts. This personalization uses individual booking data to optimize pricing strategies and improve sales.
- Ride-sharing services. Ride-sharing platforms such as Uber and Lyft use surge pricing, a form of personalized pricing, to adjust fares based on real-time demand and supply. During periods of high demand, such as rush hour or special events, prices surge significantly to encourage the availability of more drivers and ensure rides for all customers despite the increased demand.
- Personalized subscription pricing. Streaming services such as Spotify or Netflix sometimes offer personalized subscription rates to new users based on their usage patterns or regional data. For example, a user who shows interest in premium features but hasn't yet subscribed receives a limited time offer for a reduced subscription price, tailored specifically to entice them into upgrading based on their observed usage.
- E-commerce retail pricing. Online retailers such as Amazon frequently use personalized and dynamic pricing to maximize profits and stay competitive. Product prices change multiple times a day based on algorithms that consider competitor pricing, inventory levels and consumer browsing behavior, including a willingness to pay more for immediate product possession.
Differences between personalized pricing and dynamic pricing
Personalized pricing and dynamic pricing are related strategies, yet distinctive in their approaches and objectives:
- Target audience. Personalized pricing focuses on individual customers, tailoring prices based on personal data and characteristics. Dynamic pricing adjusts prices based on broader market factors such as demand fluctuations and competitor pricing.
- Data application. Personalized pricing relies heavily on customer data, including browsing history, purchase history and demographic information. Dynamic pricing primarily uses external data such as supply and demand, weather conditions and events.
- Pricing flexibility. Personalized pricing offers a high degree of flexibility by catering to individual preferences and willingness to pay. Dynamic pricing adapts to changing market conditions to maintain competitiveness.
- Use cases. Personalized pricing is common in e-commerce, subscriptions and services with direct customer interactions. Dynamic pricing is prevalent in travel, hospitality and retail, where external factors influence pricing decisions.
Benefits of personalized pricing
Personalized pricing delivers key advantages to both business and consumer, enhancing the former's overall market efficiency and the latter's overall satisfaction:
- Increased revenue optimization. Businesses maximize revenue by setting prices based on individual willingness to pay, ensuring each customer is charged the highest acceptable price.
- Enhanced customer experience. By tailoring offers and prices to individual preferences and behaviors, personalized pricing creates a more engaging and relevant shopping experience.
- Improved customer loyalty. Personalized discounts and offers strengthen customer relationships, encouraging repeat purchases and fostering long-term loyalty.
- Better competitive positioning. A business differentiates itself by offering tailored pricing that meets the specific needs of its target audience, lifting the business above competitors saddled with uniform pricing.
- Efficient inventory management. By offering discounts to customers who are most likely to purchase, personalized pricing helps businesses move inventory, reducing excess stock and minimizing waste.
Ethical considerations with personalized pricing
Personalized pricing also introduces ethical challenges that businesses must navigate transparently to maintain consumer trust and credibility.
A primary concern is privacy. The use of collected customer data to tailor prices makes some consumers uneasy, particularly those who feel their personal information is misused or even excessively monitored. To address this, savvy businesses emphasize data security and remain open with their data practices.
Fairness is another critical issue because personalized pricing creates perceptions of inequality among customers. Individuals who discover others pay different prices for the same product or service often feel a sense of unfairness and mistrust. Businesses should ensure pricing strategies do not inadvertently discriminate against certain groups and that all customers are treated equitably.
Transparency mitigates these concerns. Customers properly expect clear information about how their data is used and what factors influence the personalized prices they receive. Open communication about these practices builds trust and combats negative perceptions associated with personalized pricing.
Finally, businesses must comply with relevant regulations, such as the General Data Protection Regulation. Adhering to these laws not only avoids legal repercussions but also reinforces a company's commitment to ethical practices.
Balancing the financial benefits of personalized pricing with these ethical considerations requires a commitment to responsible data use and a focus on consumer welfare. By prioritizing transparency, fairness and regulatory compliance, businesses harness the many advantages of personalized pricing while fostering the trust of their customers.
Griffin LaFleur is a MarketingOps and RevOps professional working for Swing Education. Throughout his career, Griffin has also worked at agencies and independently as a B2B sales and marketing consultant.