complex event processing (CEP)
What is complex event processing (CEP)?
Complex event processing (CEP) is the use of technology to predict high-level events. It is a method of tracking and analyzing streams of data to gain real-time information about events.
An event, for context, is any identifiable occurrence that has significance for hardware or software. As an example, an event can be a mouse click, a program loading or a program encountering an error.
The goal of CEP is to identify meaningful events -- like potential software threats -- in real time, enabling organizations to respond to them as quickly as possible. By identifying and analyzing cause-and-effect relationships among events in real time, CEP allows personnel to take effective actions in response to specific opportunities or threats.
To identify opportunities or threats, CEP processes multiple data streams from events, discovering associations between seemingly unrelated events.
The concept and techniques of CEP were developed in the 1990s. The approach gained popularity in the heyday of service-oriented architectures (SOA), which have since been eclipsed by cloud-based architectures. Likewise, CEP has been somewhat overshadowed by event stream processing (ESP) and streaming analytics -- two approaches for processing and analyzing streams of data that implement some, but not all, of the core ideas of CEP.
However, CEP remains relevant for improving enterprise architectures and for its application in business process management, which aims to improve business processes end to end.
Business process modeling software (BPMS) tools have also started to add new functionality that lets users handle CEP, making it easier to connect to multiple sources of enterprise data. This new CEP functionality in BPMS tools enables business process managers and business owners to identify events or developments that trigger critical notifications or actions that are generally not possible to identify with enterprise software tools for customer relationship management (CRM) and enterprise resource planning (ERP).
A good example of a CEP use case of this type is an enterprise software vendor with a large, important customer coming up for renewal. The customer is experiencing a service disruption that only the customer service department is aware of -- putting the renewal at risk. CEP can put both pieces of information together -- up for renewal and service disruption -- prompting the system to trigger a notification and launch a set of activities that prioritize account servicing for this customer.
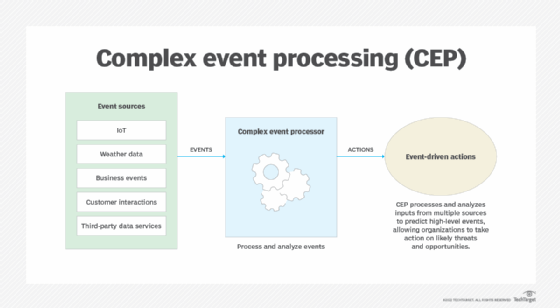
History of complex event processing (CEP)
The history of CEP begins with work done by Professor David Luckham at Stanford University.
In the 1990s, Luckham was working on distributed programming languages that could run in parallel and communicate by means of events.
"It became clear that you needed to abstract low-level events into higher-level events and do that two or three times to create accurate simulations," said Luckham, now a professor emeritus at Stanford, in an interview with TechTarget.
For example, Intel came to Luckham's team when it was trying to figure out why the adder on a new chip did not work correctly. Initially, the company thought the simulation library was deficient, but it turned out the analysis of the results lacked the ability to make sense of the raw data streams.
"In those days, simulation outputs were eyeballed by humans as streams of ones and zeros, and it was easy to miss something at that level," Luckham said. He invented the term complex events to characterize higher-level events correlated from a series of lower-level events.
Luckham's team outlined three core principles of CEP: synchronization of the timing of events, event hierarchies and causation.
- Synchronization relates to the observation that the timings of events often need to be calibrated owing to differences in processing time, network routes and latency.
- Event hierarchies relate to the development of models for expressing lower-level events, such as how clicks can translate into higher-level events like user journeys. Researchers today are developing tools for creating synthetic sensors that detect behavioral events by fusing data from various sensors.
- Causation provides a framework for connecting the dots between cause-and-effect relationships buried within a stream of events.
Luckham observed that most tools have focused on synchronization. However, more work will be required to weave the science behind event hierarchies and causation into modern tools to take advantage of the original promise of CEP.
Meanwhile, CEP's techniques to identify, analyze and process data in real time have become fundamental to many business projects.
"Today, many businesses plan strategic initiatives under titles such as 'Business Analytics' and 'Optimization.' Although they may not know it, complex event processing is usually a cornerstone of such initiatives," Luckham said.
Benefits of complex event processing
CEP's ability to detect complex patterns in multiple sources of data provides many benefits, including the following:
- Makes it easier to understand the relationship between high-level or complex events.
- Helps connect individual events into more complex chains.
- Simplifies the development and tuning of business logic.
- Can be embedded into fraud detection, logistics and internet of things (IoT) applications.
- Helps build more accurate simulations, models and predictive analytics.
- Helps improve the time to react to potentially damaging events.
- Helps recognize patterns in streamed data collection processes.
Use cases for complex event processing
The ways in which organizations use CEP today include the following:
- Improve stock market trading algorithms.
- Develop more responsive real-time marketing.
- Create more adaptable and accurate predictive maintenance.
- Detect fraud.
- Develop more nuanced IoT.
- Enable more resilient and flexible supply chains and logistics.
- Use with operational intelligence (OI) products to correlate data streams against historical data for insight.
With the growth of cloud architectures, new terms like event stream processing (ESP), real-time stream processing and streaming architectures are starting to replace the use of the term CEP in many technical discussions and product marketing.
Complex event processing as a mathematical concept is still valid, but the term itself is not used often. It might still be used in reference to temporal pattern detection -- finding new occurrences of a pattern in streaming data -- and to stream data integration, which is the loading of streaming data into databases.
The disuse of the term is due in part to how complex events are defined. For instance, events based on arithmetic aggregation operators (count the number of tweets in the last 10 minutes on "X" or topic, for example) are often not called complex events. They're relatively simple to compute, so the software does not need to be as sophisticated as in CEP.
"The technology of CEP might also be generally referred to as stream analytics or streaming analytics. Streaming analytics is widely practiced, as some organizations need to extract near-real-time insights from the vast amount of streaming data that flows through their networks.
Sophisticated forms of stream analytics, such as pattern detection, are implemented in other event stream processing platforms like Apache Flink, Spark Streaming and Tibco StreamBase.
The stream processing characteristic of CEP is also sometimes manually coded into SaaS, packaged applications or other tools, so the product implements the same math as CEP without using a CEP/ESP platform.
Still, most of the early CEP tools are still offered -- generally under the banner of stream analytics -- and remain viable. However, newer event stream processing platforms such as Microsoft Azure Stream Analytics and open source ESP platforms like Flink, Spark Streaming and Kafka Streams have taken over the bulk of new applications.
Difference between complex event processing and event stream processing
CEP is a more advanced version of event stream processing. Like CEP, ESP operates in real time and provides insights into data streams.
The difference is, however, that ESP processes events in the order they arrive, whereas CEP can process multiple events from multiple incoming data points that are taking place at the same time.
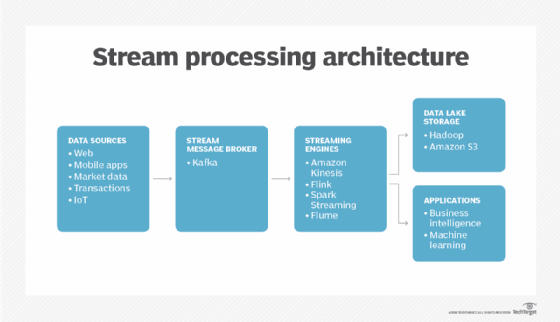
Popular tools used for complex event processing
Some popular data streaming platforms used for CEP include the following:
- Amazon Kinesis.
- Apache Kafka.
- IBM Streams.
- IBM Operational Decision Manager [ODM].
- InRule.
- Microsoft Azure Stream Analytics.
- Microsoft StreamInsight.
- Oracle Stream Analytics.
- Oracle Stream Explorer.
Amazon Kinesis, for example, is designed to detect, process and analyze real-time data streams for event analytics. It offers features such as Kinesis Data Streams for data streaming video; Kinesis Data Firehose for storing streamed data to data lakes, data sources and analytics services; and Kinesis Data Analytics for analyzing large amounts of data streamed in real time.
Likewise, Apache Kafka is a distributed event streaming platform that is deployable on bare-metal hardware, virtual machines (VMs), containers and in the cloud. The tool focuses on three capabilities: to read/write event streams, to store streams of events and to process those event streams as they occur.
Learn more about Apache Kafka, its best use cases, as well as areas where the tool might not be a good fit. Explore 10 tools to level up streaming analytics platforms, four types of simulation models used in data analytics and seven enterprise use cases for real-time streaming analytics.